Topic:Visual Place Recognition
What is Visual Place Recognition? Visual place recognition is the task of matching a view of a place with a different view of the same place taken at a different time.
Papers and Code
Apr 14, 2025
Abstract:Visual Place Recognition (VPR) is aimed at predicting the location of a query image by referencing a database of geotagged images. For VPR task, often fewer discriminative local regions in an image produce important effects while mundane background regions do not contribute or even cause perceptual aliasing because of easy overlap. However, existing methods lack precisely modeling and full exploitation of these discriminative regions. In this paper, we propose the Focus on Local (FoL) approach to stimulate the performance of image retrieval and re-ranking in VPR simultaneously by mining and exploiting reliable discriminative local regions in images and introducing pseudo-correlation supervision. First, we design two losses, Extraction-Aggregation Spatial Alignment Loss (SAL) and Foreground-Background Contrast Enhancement Loss (CEL), to explicitly model reliable discriminative local regions and use them to guide the generation of global representations and efficient re-ranking. Second, we introduce a weakly-supervised local feature training strategy based on pseudo-correspondences obtained from aggregating global features to alleviate the lack of local correspondences ground truth for the VPR task. Third, we suggest an efficient re-ranking pipeline that is efficiently and precisely based on discriminative region guidance. Finally, experimental results show that our FoL achieves the state-of-the-art on multiple VPR benchmarks in both image retrieval and re-ranking stages and also significantly outperforms existing two-stage VPR methods in terms of computational efficiency. Code and models are available at https://github.com/chenshunpeng/FoL
* Accepted by AAAI 2025
Via
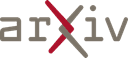
Apr 15, 2025
Abstract:The standard approach for visual place recognition is to use global image descriptors to retrieve the most similar database images for a given query image. The results can then be further improved with re-ranking methods that re-order the top scoring images. However, existing methods focus on re-ranking based on the same image descriptors that were used for the initial retrieval, which we argue provides limited additional signal. In this work we propose Generalized Contextual Similarity Aggregation (GCSA), which is a graph neural network-based re-ranking method that, in addition to the visual descriptors, can leverage other types of available side information. This can for example be other sensor data (such as signal strength of nearby WiFi or BlueTooth endpoints) or geometric properties such as camera poses for database images. In many applications this information is already present or can be acquired with low effort. Our architecture leverages the concept of affinity vectors to allow for a shared encoding of the heterogeneous multi-modal input. Two large-scale datasets, covering both outdoor and indoor localization scenarios, are utilized for training and evaluation. In experiments we show significant improvement not only on image retrieval metrics, but also for the downstream visual localization task.
* Accepted at Scandinavian Conference on Image Analysis (SCIA) 2025
Via
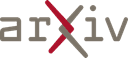
Apr 08, 2025
Abstract:Visual Place Recognition (VPR) is a critical task in computer vision, traditionally enhanced by re-ranking retrieval results with image matching. However, recent advancements in VPR methods have significantly improved performance, challenging the necessity of re-ranking. In this work, we show that modern retrieval systems often reach a point where re-ranking can degrade results, as current VPR datasets are largely saturated. We propose using image matching as a verification step to assess retrieval confidence, demonstrating that inlier counts can reliably predict when re-ranking is beneficial. Our findings shift the paradigm of retrieval pipelines, offering insights for more robust and adaptive VPR systems.
* CVPRW 2025
Via
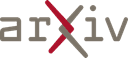
Mar 27, 2025
Abstract:Visual place recognition (VPR) is crucial for robots to identify previously visited locations, playing an important role in autonomous navigation in both indoor and outdoor environments. However, most existing VPR datasets are limited to single-viewpoint scenarios, leading to reduced recognition accuracy, particularly in multi-directional driving or feature-sparse scenes. Moreover, obtaining additional data to mitigate these limitations is often expensive. This paper introduces a novel training paradigm to improve the performance of existing VPR networks by enhancing multi-view diversity within current datasets through uncertainty estimation and NeRF-based data augmentation. Specifically, we initially train NeRF using the existing VPR dataset. Then, our devised self-supervised uncertainty estimation network identifies places with high uncertainty. The poses of these uncertain places are input into NeRF to generate new synthetic observations for further training of VPR networks. Additionally, we propose an improved storage method for efficient organization of augmented and original training data. We conducted extensive experiments on three datasets and tested three different VPR backbone networks. The results demonstrate that our proposed training paradigm significantly improves VPR performance by fully utilizing existing data, outperforming other training approaches. We further validated the effectiveness of our approach on self-recorded indoor and outdoor datasets, consistently demonstrating superior results. Our dataset and code have been released at \href{https://github.com/nubot-nudt/UGNA-VPR}{https://github.com/nubot-nudt/UGNA-VPR}.
* Accepted to IEEE Robotics and Automation Letters (RA-L)
Via
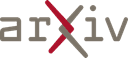
Mar 17, 2025
Abstract:Uniform and variable environments still remain a challenge for stable visual localization and mapping in mobile robot navigation. One of the possible approaches suitable for such environments is appearance-based teach-and-repeat navigation, relying on simplified localization and reactive robot motion control - all without a need for standard mapping. This work brings an innovative solution to such a system based on visual place recognition techniques. Here, the major contributions stand in the employment of a new visual place recognition technique, a novel horizontal shift computation approach, and a multi-platform system design for applications across various types of mobile robots. Secondly, a new public dataset for experimental testing of appearance-based navigation methods is introduced. Moreover, the work also provides real-world experimental testing and performance comparison of the introduced navigation system against other state-of-the-art methods. The results confirm that the new system outperforms existing methods in several testing scenarios, is capable of operation indoors and outdoors, and exhibits robustness to day and night scene variations.
* 6 pages, 5 figures
Via
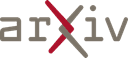
Mar 11, 2025
Abstract:Visual Place Recognition (VPR) aims to estimate the location of the given query image within a database of geo-tagged images. To identify the exact location in an image, detecting landmarks is crucial. However, in some scenarios, such as urban environments, there are numerous landmarks, such as various modern buildings, and the landmarks in different cities often exhibit high visual similarity. Therefore, it is essential not only to leverage the landmarks but also to consider the contextual information surrounding them, such as whether there are trees, roads, or other features around the landmarks. We propose the Contextual Query VPR (CQVPR), which integrates contextual information with detailed pixel-level visual features. By leveraging a set of learnable contextual queries, our method automatically learns the high-level contexts with respect to landmarks and their surrounding areas. Heatmaps depicting regions that each query attends to serve as context-aware features, offering cues that could enhance the understanding of each scene. We further propose a query matching loss to supervise the extraction process of contextual queries. Extensive experiments on several datasets demonstrate that the proposed method outperforms other state-of-the-art methods, especially in challenging scenarios.
Via
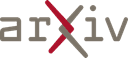
Mar 09, 2025
Abstract:Visual place recognition is a challenging task for autonomous driving and robotics, which is usually considered as an image retrieval problem. A commonly used two-stage strategy involves global retrieval followed by re-ranking using patch-level descriptors. Most deep learning-based methods in an end-to-end manner cannot extract global features with sufficient semantic information from RGB images. In contrast, re-ranking can utilize more explicit structural and semantic information in one-to-one matching process, but it is time-consuming. To bridge the gap between global retrieval and re-ranking and achieve a good trade-off between accuracy and efficiency, we propose StructVPR++, a framework that embeds structural and semantic knowledge into RGB global representations via segmentation-guided distillation. Our key innovation lies in decoupling label-specific features from global descriptors, enabling explicit semantic alignment between image pairs without requiring segmentation during deployment. Furthermore, we introduce a sample-wise weighted distillation strategy that prioritizes reliable training pairs while suppressing noisy ones. Experiments on four benchmarks demonstrate that StructVPR++ surpasses state-of-the-art global methods by 5-23% in Recall@1 and even outperforms many two-stage approaches, achieving real-time efficiency with a single RGB input.
Via
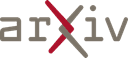
Mar 10, 2025
Abstract:In visual place recognition (VPR), filtering and sequence-based matching approaches can improve performance by integrating temporal information across image sequences, especially in challenging conditions. While these methods are commonly applied, their effects on system behavior can be unpredictable and can actually make performance worse in certain situations. In this work, we present a new supervised learning approach that learns to predict the per-frame sequence matching receptiveness (SMR) of VPR techniques, enabling the system to selectively decide when to trust the output of a sequence matching system. The approach is agnostic to the underlying VPR technique. Our approach predicts SMR-and hence significantly improves VPR performance-across a large range of state-of-the-art and classical VPR techniques (namely CosPlace, MixVPR, EigenPlaces, SALAD, AP-GeM, NetVLAD and SAD), and across three benchmark VPR datasets (Nordland, Oxford RobotCar, and SFU-Mountain). We also provide insights into a complementary approach that uses the predictor to replace discarded matches, as well as ablation studies, including an analysis of the interactions between our SMR predictor and the selected sequence length. We will release our code upon acceptance.
* 8 pages, 5 figures, under review
Via
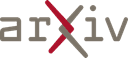
Mar 09, 2025
Abstract:Visual Place Recognition (VPR) is a crucial capability for long-term autonomous robots, enabling them to identify previously visited locations using visual information. However, existing methods remain limited in indoor settings due to the highly repetitive structures inherent in such environments. We observe that scene text typically appears in indoor spaces, serving to distinguish visually similar but different places. This inspires us to propose TextInPlace, a simple yet effective VPR framework that integrates Scene Text Spotting (STS) to mitigate visual perceptual ambiguity in repetitive indoor environments. Specifically, TextInPlace adopts a dual-branch architecture within a local parameter sharing network. The VPR branch employs attention-based aggregation to extract global descriptors for coarse-grained retrieval, while the STS branch utilizes a bridging text spotter to detect and recognize scene text. Finally, the discriminative text is filtered to compute text similarity and re-rank the top-K retrieved images. To bridge the gap between current text-based repetitive indoor scene datasets and the typical scenarios encountered in robot navigation, we establish an indoor VPR benchmark dataset, called Maze-with-Text. Extensive experiments on both custom and public datasets demonstrate that TextInPlace achieves superior performance over existing methods that rely solely on appearance information. The dataset, code, and trained models are publicly available at https://github.com/HqiTao/TextInPlace.
* 8 pages,5 figures
Via
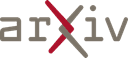
Mar 04, 2025
Abstract:Visual Place Recognition (VPR) localizes a query image by matching it against a database of geo-tagged reference images, making it essential for navigation and mapping in robotics. Although Vision Transformer (ViT) solutions deliver high accuracy, their large models often exceed the memory and compute budgets of resource-constrained platforms such as drones and mobile robots. To address this issue, we propose TeTRA, a ternary transformer approach that progressively quantizes the ViT backbone to 2-bit precision and binarizes its final embedding layer, offering substantial reductions in model size and latency. A carefully designed progressive distillation strategy preserves the representational power of a full-precision teacher, allowing TeTRA to retain or even surpass the accuracy of uncompressed convolutional counterparts, despite using fewer resources. Experiments on standard VPR benchmarks demonstrate that TeTRA reduces memory consumption by up to 69% compared to efficient baselines, while lowering inference latency by 35%, with either no loss or a slight improvement in recall@1. These gains enable high-accuracy VPR on power-constrained, memory-limited robotic platforms, making TeTRA an appealing solution for real-world deployment.
Via
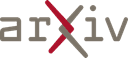