Topic:Multiple Instance Learning
What is Multiple Instance Learning? Multiple instance learning is a machine learning paradigm where training data is organized into bags of instances.
Papers and Code
Apr 24, 2025
Abstract:Multiple instance learning (MIL) is a promising approach for weakly supervised classification in pathology using whole slide images (WSIs). However, conventional MIL methods such as Attention-Based Deep Multiple Instance Learning (ABMIL) typically disregard spatial interactions among patches that are crucial to pathological diagnosis. Recent advancements, such as Transformer based MIL (TransMIL), have incorporated spatial context and inter-patch relationships. However, it remains unclear whether explicitly modeling patch relationships yields similar performance gains in ABMIL, which relies solely on Multi-Layer Perceptrons (MLPs). In contrast, TransMIL employs Transformer-based layers, introducing a fundamental architectural shift at the cost of substantially increased computational complexity. In this work, we enhance the ABMIL framework by integrating interaction-aware representations to address this question. Our proposed model, Global ABMIL (GABMIL), explicitly captures inter-instance dependencies while preserving computational efficiency. Experimental results on two publicly available datasets for tumor subtyping in breast and lung cancers demonstrate that GABMIL achieves up to a 7 percentage point improvement in AUPRC and a 5 percentage point increase in the Kappa score over ABMIL, with minimal or no additional computational overhead. These findings underscore the importance of incorporating patch interactions within MIL frameworks.
Via
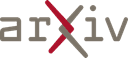
Apr 24, 2025
Abstract:The Contrastive Language-Image Pre-training (CLIP) framework has become a widely used approach for multimodal representation learning, particularly in image-text retrieval and clustering. However, its efficacy is constrained by three key limitations: (1) text token truncation, (2) isolated image-text encoding, and (3) deficient compositionality due to bag-of-words behavior. While recent Multimodal Large Language Models (MLLMs) have demonstrated significant advances in generalized vision-language understanding, their potential for learning transferable multimodal representations remains underexplored.In this work, we present UniME (Universal Multimodal Embedding), a novel two-stage framework that leverages MLLMs to learn discriminative representations for diverse downstream tasks. In the first stage, we perform textual discriminative knowledge distillation from a powerful LLM-based teacher model to enhance the embedding capability of the MLLM\'s language component. In the second stage, we introduce hard negative enhanced instruction tuning to further advance discriminative representation learning. Specifically, we initially mitigate false negative contamination and then sample multiple hard negatives per instance within each batch, forcing the model to focus on challenging samples. This approach not only improves discriminative power but also enhances instruction-following ability in downstream tasks. We conduct extensive experiments on the MMEB benchmark and multiple retrieval tasks, including short and long caption retrieval and compositional retrieval. Results demonstrate that UniME achieves consistent performance improvement across all tasks, exhibiting superior discriminative and compositional capabilities.
* 13 pages, 8 figures, Project page: https://garygutc.github.io/UniME
Via
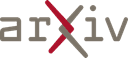
Apr 21, 2025
Abstract:Multiple Instance Learning (MIL) is a popular weakly-supervised method for various applications, with a particular interest in histological whole slide image (WSI) classification. Due to the gigapixel resolution of WSI, applications of MIL in WSI typically necessitate a two-stage training scheme: first, extract features from the pre-trained backbone and then perform MIL aggregation. However, it is well-known that this suboptimal training scheme suffers from "noisy" feature embeddings from the backbone and inherent weak supervision, hindering MIL from learning rich and generalizable features. However, the most commonly used technique (i.e., dropout) for mitigating this issue has yet to be explored in MIL. In this paper, we empirically explore how effective the dropout can be in MIL. Interestingly, we observe that dropping the top-k most important instances within a bag leads to better performance and generalization even under noise attack. Based on this key observation, we propose a novel MIL-specific dropout method, termed MIL-Dropout, which systematically determines which instances to drop. Experiments on five MIL benchmark datasets and two WSI datasets demonstrate that MIL-Dropout boosts the performance of current MIL methods with a negligible computational cost. The code is available at https://github.com/ChongQingNoSubway/MILDropout.
Via
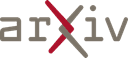
Apr 21, 2025
Abstract:Graph Coloring Problem (GCP) is an NP-Hard vertex labeling problem in graphs such that no two adjacent vertices can have the same color. Large instances of GCP cannot be solved in reasonable execution times by exact algorithms. Therefore, soft computing approaches, such as metaheuristics, have proven to be very efficient for solving large instances of GCP. In this study, we propose a new island-parallel ensemble metaheuristic algorithm (PEM-Color) to solve large GCP instances. Ensemble learning is a new machine learning approach based on combining the output of multiple models instead of using a single one. We use Message Passing Interface (MPI) parallel computation libraries to combine recent state-of-the-art metaheuristics: Harris Hawk Optimization (HHO), Artificial Bee Colony (ABC), and Teaching Learning Based (TLBO) to improve the quality of their solutions further. To the best of our knowledge, this is the first study that combines metaheuristics and applies to the GCP using an ensemble approach. We conducted experiments on large graph instances from the well-known DIMACS benchmark using 64 processors and achieved significant improvements in execution times. The experiments also indicate an almost linear speed-up with a strong scalability potential. The solution quality of the instances is promising, as our algorithm outperforms 13 state-of-the-art algorithms.
* 29 pages, 4 Tables, 5 algorithms, 4 figures
Via
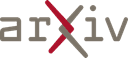
Apr 18, 2025
Abstract:Cytology is essential for cancer diagnostics and screening due to its minimally invasive nature. However, the development of robust deep learning models for digital cytology is challenging due to the heterogeneity in staining and preparation methods of samples, differences across organs, and the limited availability of large, diverse, annotated datasets. Developing a task-specific model for every cytology application is impractical and non-cytology-specific foundation models struggle to generalize to tasks in this domain where the emphasis is on cell morphology. To address these challenges, we introduce CytoFM, the first cytology self-supervised foundation model. Using iBOT, a self-supervised Vision Transformer (ViT) training framework incorporating masked image modeling and self-distillation, we pretrain CytoFM on a diverse collection of cytology datasets to learn robust, transferable representations. We evaluate CytoFM on multiple downstream cytology tasks, including breast cancer classification and cell type identification, using an attention-based multiple instance learning framework. Our results demonstrate that CytoFM performs better on two out of three downstream tasks than existing foundation models pretrained on histopathology (UNI) or natural images (iBOT-Imagenet). Visualizations of learned representations demonstrate our model is able to attend to cytologically relevant features. Despite a small pre-training dataset, CytoFM's promising results highlight the ability of task-agnostic pre-training approaches to learn robust and generalizable features from cytology data.
Via
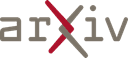
Apr 21, 2025
Abstract:We present Distributional RewArds for Generative OptimizatioN (DRAGON), a versatile framework for fine-tuning media generation models towards a desired outcome. Compared with traditional reinforcement learning with human feedback (RLHF) or pairwise preference approaches such as direct preference optimization (DPO), DRAGON is more flexible. It can optimize reward functions that evaluate either individual examples or distributions of them, making it compatible with a broad spectrum of instance-wise, instance-to-distribution, and distribution-to-distribution rewards. Leveraging this versatility, we construct novel reward functions by selecting an encoder and a set of reference examples to create an exemplar distribution. When cross-modality encoders such as CLAP are used, the reference examples may be of a different modality (e.g., text versus audio). Then, DRAGON gathers online and on-policy generations, scores them to construct a positive demonstration set and a negative set, and leverages the contrast between the two sets to maximize the reward. For evaluation, we fine-tune an audio-domain text-to-music diffusion model with 20 different reward functions, including a custom music aesthetics model, CLAP score, Vendi diversity, and Frechet audio distance (FAD). We further compare instance-wise (per-song) and full-dataset FAD settings while ablating multiple FAD encoders and reference sets. Over all 20 target rewards, DRAGON achieves an 81.45% average win rate. Moreover, reward functions based on exemplar sets indeed enhance generations and are comparable to model-based rewards. With an appropriate exemplar set, DRAGON achieves a 60.95% human-voted music quality win rate without training on human preference annotations. As such, DRAGON exhibits a new approach to designing and optimizing reward functions for improving human-perceived quality. Sound examples at https://ml-dragon.github.io/web.
Via
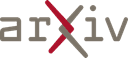
Apr 16, 2025
Abstract:Computer-aided Whole Slide Image (WSI) classification has the potential to enhance the accuracy and efficiency of clinical pathological diagnosis. It is commonly formulated as a Multiple Instance Learning (MIL) problem, where each WSI is treated as a bag and the small patches extracted from the WSI are considered instances within that bag. However, obtaining labels for a large number of bags is a costly and time-consuming process, particularly when utilizing existing WSIs for new classification tasks. This limitation renders most existing WSI classification methods ineffective. To address this issue, we propose a novel WSI classification problem setting, more aligned with clinical practice, termed Weakly Semi-supervised Whole slide image Classification (WSWC). In WSWC, a small number of bags are labeled, while a significant number of bags remain unlabeled. The MIL nature of the WSWC problem, coupled with the absence of patch labels, distinguishes it from typical semi-supervised image classification problems, making existing algorithms for natural images unsuitable for directly solving the WSWC problem. In this paper, we present a concise and efficient framework, named CroCo, to tackle the WSWC problem through two-level Cross Consistency supervision. CroCo comprises two heterogeneous classifier branches capable of performing both instance classification and bag classification. The fundamental idea is to establish cross-consistency supervision at both the bag-level and instance-level between the two branches during training. Extensive experiments conducted on four datasets demonstrate that CroCo achieves superior bag classification and instance classification performance compared to other comparative methods when limited WSIs with bag labels are available. To the best of our knowledge, this paper presents for the first time the WSWC problem and gives a successful resolution.
Via
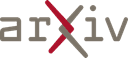
Apr 13, 2025
Abstract:Inflammatory bowel disease (IBD) involves chronic inflammation of the digestive tract, with treatment options often burdened by adverse effects. Identifying biomarkers for personalized treatment is crucial. While immune cells play a key role in IBD, accurately identifying ulcer regions in whole slide images (WSIs) is essential for characterizing these cells and exploring potential therapeutics. Multiple instance learning (MIL) approaches have advanced WSI analysis but they lack spatial context awareness. In this work, we propose a weakly-supervised model called DomainGCN that employs a graph convolution neural network (GCN) and incorporates domain-specific knowledge of ulcer features, specifically, the presence of epithelium, lymphocytes, and debris for WSI-level ulcer prediction in IBD. We demonstrate that DomainGCN outperforms various state-of-the-art (SOTA) MIL methods and show the added value of domain knowledge.
* Work accepted at ISBI 2025
Via
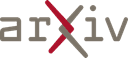
Apr 15, 2025
Abstract:Bipartite ranking is a fundamental supervised learning problem, with the goal of learning a ranking over instances with maximal area under the ROC curve (AUC) against a single binary target label. However, one may often observe multiple binary target labels, e.g., from distinct human annotators. How can one synthesize such labels into a single coherent ranking? In this work, we formally analyze two approaches to this problem -- loss aggregation and label aggregation -- by characterizing their Bayes-optimal solutions. Based on this, we show that while both methods can yield Pareto-optimal solutions, loss aggregation can exhibit label dictatorship: one can inadvertently (and undesirably) favor one label over others. This suggests that label aggregation can be preferable to loss aggregation, which we empirically verify.
Via
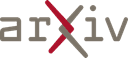
Apr 16, 2025
Abstract:We propose CAL (Complete Anything in Lidar) for Lidar-based shape-completion in-the-wild. This is closely related to Lidar-based semantic/panoptic scene completion. However, contemporary methods can only complete and recognize objects from a closed vocabulary labeled in existing Lidar datasets. Different to that, our zero-shot approach leverages the temporal context from multi-modal sensor sequences to mine object shapes and semantic features of observed objects. These are then distilled into a Lidar-only instance-level completion and recognition model. Although we only mine partial shape completions, we find that our distilled model learns to infer full object shapes from multiple such partial observations across the dataset. We show that our model can be prompted on standard benchmarks for Semantic and Panoptic Scene Completion, localize objects as (amodal) 3D bounding boxes, and recognize objects beyond fixed class vocabularies. Our project page is https://research.nvidia.com/labs/dvl/projects/complete-anything-lidar
Via
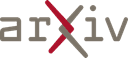