What is Few Shot Learning? Few-shot learning is a machine-learning paradigm where models are trained with limited labeled data.
Papers and Code
Dec 24, 2024
Abstract:We introduce a neural network conformal prediction method for time series that enhances adaptivity in non-stationary environments. Our approach acts as a neural controller designed to achieve desired target coverage, leveraging auxiliary multi-view data with neural network encoders in an end-to-end manner to further enhance adaptivity. Additionally, our model is designed to enhance the consistency of prediction intervals in different quantiles by integrating monotonicity constraints and leverages data from related tasks to boost few-shot learning performance. Using real-world datasets from epidemics, electric demand, weather, and others, we empirically demonstrate significant improvements in coverage and probabilistic accuracy, and find that our method is the only one that combines good calibration with consistency in prediction intervals.
Via
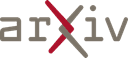
Dec 24, 2024
Abstract:We introduce Switch-a-View, a model that learns to automatically select the viewpoint to display at each timepoint when creating a how-to video. The key insight of our approach is how to train such a model from unlabeled--but human-edited--video samples. We pose a pretext task that pseudo-labels segments in the training videos for their primary viewpoint (egocentric or exocentric), and then discovers the patterns between those view-switch moments on the one hand and the visual and spoken content in the how-to video on the other hand. Armed with this predictor, our model then takes an unseen multi-view video as input and orchestrates which viewpoint should be displayed when. We further introduce a few-shot training setting that permits steering the model towards a new data domain. We demonstrate our idea on a variety of real-world video from HowTo100M and Ego-Exo4D and rigorously validate its advantages.
Via
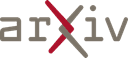
Dec 24, 2024
Abstract:Recent advances in flexible keyword spotting (KWS) with text enrollment allow users to personalize keywords without uttering them during enrollment. However, there is still room for improvement in target keyword performance. In this work, we propose a novel few-shot transfer learning method, called text-aware adapter (TA-adapter), designed to enhance a pre-trained flexible KWS model for specific keywords with limited speech samples. To adapt the acoustic encoder, we leverage a jointly pre-trained text encoder to generate a text embedding that acts as a representative vector for the keyword. By fine-tuning only a small portion of the network while keeping the core components' weights intact, the TA-adapter proves highly efficient for few-shot KWS, enabling a seamless return to the original pre-trained model. In our experiments, the TA-adapter demonstrated significant performance improvements across 35 distinct keywords from the Google Speech Commands V2 dataset, with only a 0.14% increase in the total number of parameters.
* 5 pages, 3 figures, Accepted by ICASSP 2025
Via
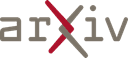
Dec 23, 2024
Abstract:Retrieval augmentation, the practice of retrieving additional data from large auxiliary pools, has emerged as an effective technique for enhancing model performance in the low-data regime, e.g. few-shot learning. Prior approaches have employed only nearest-neighbor based strategies for data selection, which retrieve auxiliary samples with high similarity to instances in the target task. However, these approaches are prone to selecting highly redundant samples, since they fail to incorporate any notion of diversity. In our work, we first demonstrate that data selection strategies used in prior retrieval-augmented few-shot learning settings can be generalized using a class of functions known as Combinatorial Mutual Information (CMI) measures. We then propose COBRA (COmBinatorial Retrieval Augmentation), which employs an alternative CMI measure that considers both diversity and similarity to a target dataset. COBRA consistently outperforms previous retrieval approaches across image classification tasks and few-shot learning techniques when used to retrieve samples from LAION-2B. COBRA introduces negligible computational overhead to the cost of retrieval while providing significant gains in downstream model performance.
Via
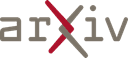
Dec 23, 2024
Abstract:Generative Zero-Shot Learning (ZSL) methods synthesize class-related features based on predefined class semantic prototypes, showcasing superior performance. However, this feature generation paradigm falls short of providing interpretable insights. In addition, existing approaches rely on semantic prototypes annotated by human experts, which exhibit a significant limitation in their scalability to generalized scenes. To overcome these deficiencies, a natural solution is to generate images for unseen classes using text prompts. To this end, We present DIG-ZSL, a novel Discriminative Image Generation framework for Zero-Shot Learning. Specifically, to ensure the generation of discriminative images for training an effective ZSL classifier, we learn a discriminative class token (DCT) for each unseen class under the guidance of a pre-trained category discrimination model (CDM). Harnessing DCTs, we can generate diverse and high-quality images, which serve as informative unseen samples for ZSL tasks. In this paper, the extensive experiments and visualizations on four datasets show that our DIG-ZSL: (1) generates diverse and high-quality images, (2) outperforms previous state-of-the-art nonhuman-annotated semantic prototype-based methods by a large margin, and (3) achieves comparable or better performance than baselines that leverage human-annotated semantic prototypes. The codes will be made available upon acceptance of the paper.
* Tech report, 16 pages
Via
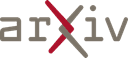
Dec 23, 2024
Abstract:Few-shot learning aims to recognize novel concepts by leveraging prior knowledge learned from a few samples. However, for visually intensive tasks such as few-shot semantic segmentation, pixel-level annotations are time-consuming and costly. Therefore, in this paper, we utilize the more challenging image-level annotations and propose an adaptive frequency-aware network (AFANet) for weakly-supervised few-shot semantic segmentation (WFSS). Specifically, we first propose a cross-granularity frequency-aware module (CFM) that decouples RGB images into high-frequency and low-frequency distributions and further optimizes semantic structural information by realigning them. Unlike most existing WFSS methods using the textual information from the multi-modal language-vision model, e.g., CLIP, in an offline learning manner, we further propose a CLIP-guided spatial-adapter module (CSM), which performs spatial domain adaptive transformation on textual information through online learning, thus providing enriched cross-modal semantic information for CFM. Extensive experiments on the Pascal-5\textsuperscript{i} and COCO-20\textsuperscript{i} datasets demonstrate that AFANet has achieved state-of-the-art performance. The code is available at https://github.com/jarch-ma/AFANet.
Via
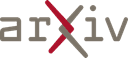
Dec 24, 2024
Abstract:Computer Vision (CV) has yet to fully achieve the zero-shot task generalization observed in Natural Language Processing (NLP), despite following many of the milestones established in NLP, such as large transformer models, extensive pre-training, and the auto-regression paradigm, among others. In this paper, we explore the idea that CV adopts discrete and terminological task definitions (\eg, ``image segmentation''), which may be a key barrier to zero-shot task generalization. Our hypothesis is that without truly understanding previously-seen tasks--due to these terminological definitions--deep models struggle to generalize to novel tasks. To verify this, we introduce Explanatory Instructions, which provide an intuitive way to define CV task objectives through detailed linguistic transformations from input images to outputs. We create a large-scale dataset comprising 12 million ``image input $\to$ explanatory instruction $\to$ output'' triplets, and train an auto-regressive-based vision-language model (AR-based VLM) that takes both images and explanatory instructions as input. By learning to follow these instructions, the AR-based VLM achieves instruction-level zero-shot capabilities for previously-seen tasks and demonstrates strong zero-shot generalization for unseen CV tasks. Code and dataset will be openly available on our GitHub repository.
* 40 pages
Via
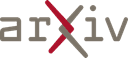
Dec 23, 2024
Abstract:Causal reasoning capabilities are essential for large language models (LLMs) in a wide range of applications, such as education and healthcare. But there is still a lack of benchmarks for a better understanding of such capabilities. Current LLM benchmarks are mainly based on conversational tasks, academic math tests, and coding tests. Such benchmarks evaluate LLMs in well-regularized settings, but they are limited in assessing the skills and abilities to solve real-world problems. In this work, we provide a benchmark, named by CARL-GT, which evaluates CAusal Reasoning capabilities of large Language models using Graphs and Tabular data. The benchmark has a diverse range of tasks for evaluating LLMs from causal graph reasoning, knowledge discovery, and decision-making aspects. In addition, effective zero-shot learning prompts are developed for the tasks. In our experiments, we leverage the benchmark for evaluating open-source LLMs and provide a detailed comparison of LLMs for causal reasoning abilities. We found that LLMs are still weak in casual reasoning, especially with tabular data to discover new insights. Furthermore, we investigate and discuss the relationships of different benchmark tasks by analyzing the performance of LLMs. The experimental results show that LLMs have different strength over different tasks and that their performance on tasks in different categories, i.e., causal graph reasoning, knowledge discovery, and decision-making, shows stronger correlation than tasks in the same category.
Via
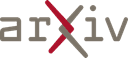
Dec 24, 2024
Abstract:Large Language Models (LLMs) excel in linguistic tasks but struggle with mathematical reasoning, particularly in non English languages like Hindi. This research aims to enhance the mathematical reasoning skills of smaller, resource efficient open-source LLMs in both Hindi and English. We evaluate models like OpenHathi 7B, LLaMA-2 7B, WizardMath 7B, Mistral 7B, LLeMMa 7B, MAmmoTH 7B, Gemini Pro, and GPT-4 using zero-shot, few-shot chain-of-thought (CoT) methods, and supervised fine-tuning. Our approach incorporates curriculum learning, progressively training models on increasingly difficult problems, a novel Decomposition Strategy to simplify complex arithmetic operations, and a Structured Solution Design that divides solutions into phases. Our experiments result in notable performance enhancements. WizardMath 7B exceeds Gemini's accuracy on English datasets by +6% and matches Gemini's performance on Hindi datasets. Adopting a bilingual approach that combines English and Hindi samples achieves results comparable to individual language models, demonstrating the capability to learn mathematical reasoning in both languages. This research highlights the potential for improving mathematical reasoning in open-source LLMs.
* Accepted at AAAI 2025
Via
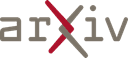
Dec 24, 2024
Abstract:The item cold-start problem is crucial for online recommender systems, as the success of the cold-start phase determines whether items can transition into popular ones. Prompt learning, a powerful technique used in natural language processing (NLP) to address zero- or few-shot problems, has been adapted for recommender systems to tackle similar challenges. However, existing methods typically rely on content-based properties or text descriptions for prompting, which we argue may be suboptimal for cold-start recommendations due to 1) semantic gaps with recommender tasks, 2) model bias caused by warm-up items contribute most of the positive feedback to the model, which is the core of the cold-start problem that hinders the recommender quality on cold-start items. We propose to leverage high-value positive feedback, termed pinnacle feedback as prompt information, to simultaneously resolve the above two problems. We experimentally prove that compared to the content description proposed in existing works, the positive feedback is more suitable to serve as prompt information by bridging the semantic gaps. Besides, we propose item-wise personalized prompt networks to encode pinnaclce feedback to relieve the model bias by the positive feedback dominance problem. Extensive experiments on four real-world datasets demonstrate the superiority of our model over state-of-the-art methods. Moreover, PROMO has been successfully deployed on a popular short-video sharing platform, a billion-user scale commercial short-video application, achieving remarkable performance gains across various commercial metrics within cold-start scenarios
Via
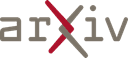