What is Head Detection? Head detection is the process of identifying and locating human heads in images or videos.
Papers and Code
Apr 24, 2025
Abstract:As false information continues to proliferate across social media platforms, effective rumor detection has emerged as a pressing challenge in natural language processing. This paper proposes RAGAT-Mind, a multi-granular modeling approach for Chinese rumor detection, built upon the MindSpore deep learning framework. The model integrates TextCNN for local semantic extraction, bidirectional GRU for sequential context learning, Multi-Head Self-Attention for global dependency focusing, and Bidirectional Graph Convolutional Networks (BiGCN) for structural representation of word co-occurrence graphs. Experiments on the Weibo1-Rumor dataset demonstrate that RAGAT-Mind achieves superior classification performance, attaining 99.2% accuracy and a macro-F1 score of 0.9919. The results validate the effectiveness of combining hierarchical linguistic features with graph-based semantic structures. Furthermore, the model exhibits strong generalization and interpretability, highlighting its practical value for real-world rumor detection applications.
Via
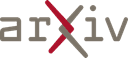
Apr 24, 2025
Abstract:The escalating overlap between non-geostationary orbit (NGSO) and geostationary orbit (GSO) satellite frequency allocations necessitates accurate interference detection methods that address two pivotal technical gaps: computationally efficient signal analysis for real-time operation, and robust anomaly discrimination under varying interference patterns. Existing deep learning approaches employ encoder-decoder anomaly detectors that threshold input-output discrepancies for robustness. While the transformer-based TrID model achieves state-of-the-art performance (AUC: 0.8318, F1: 0.8321), its multi-head attention incurs prohibitive computation time, and its decoupled training of time-frequency models overlooks cross-domain dependencies. To overcome these problems, we propose DualAttWaveNet. A bidirectional attention fusion layer dynamically correlates time-domain samples using parameter-efficient cross-attention routing. A wavelet-regularized reconstruction loss enforces multi-scale consistency. We train the model on public dataset which consists of 48 hours of satellite signals. Experiments show that compared to TrID, DualAttWaveNet improves AUC by 12% and reduces inference time by 50% to 540ms per batch while maintaining F1-score.
Via
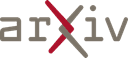
Apr 22, 2025
Abstract:In medical imaging, anomaly detection is a vital element of healthcare diagnostics, especially for neurological conditions which can be life-threatening. Conventional deterministic methods often fall short when it comes to capturing the inherent uncertainty of anomaly detection tasks. This paper introduces a Bayesian Variational Autoencoder (VAE) equipped with multi-head attention mechanisms for detecting anomalies in brain magnetic resonance imaging (MRI). For the purpose of improving anomaly detection performance, we incorporate both epistemic and aleatoric uncertainty estimation through Bayesian inference. The model was tested on the BraTS2020 dataset, and the findings were a 0.83 ROC AUC and a 0.83 PR AUC. The data in our paper suggests that modeling uncertainty is an essential component of anomaly detection, enhancing both performance and interpretability and providing confidence estimates, as well as anomaly predictions, for clinicians to leverage in making medical decisions.
* 16 pages, 6 figures
Via
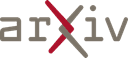
Apr 22, 2025
Abstract:Imaging the human body's morphological and angiographic information is essential for diagnosing, monitoring, and treating medical conditions. Ultrasonography performs the morphological assessment of the soft tissue based on acoustic impedance variations, whereas photoacoustic tomography (PAT) can visualize blood vessels based on intrinsic hemoglobin absorption. Three-dimensional (3D) panoramic imaging of the vasculature is generally not practical in conventional ultrasonography with limited field-of-view (FOV) probes, and PAT does not provide sufficient scattering-based soft tissue morphological contrast. Complementing each other, fast panoramic rotational ultrasound tomography (RUST) and PAT are integrated for hybrid rotational ultrasound and photoacoustic tomography (RUS-PAT), which obtains 3D ultrasound structural and PAT angiographic images of the human body quasi-simultaneously. The RUST functionality is achieved in a cost-effective manner using a single-element ultrasonic transducer for ultrasound transmission and rotating arc-shaped arrays for 3D panoramic detection. RUST is superior to conventional ultrasonography, which either has a limited FOV with a linear array or is high-cost with a hemispherical array that requires both transmission and receiving. By switching the acoustic source to a light source, the system is conveniently converted to PAT mode to acquire angiographic images in the same region. Using RUS-PAT, we have successfully imaged the human head, breast, hand, and foot with a 10 cm diameter FOV, submillimeter isotropic resolution, and 10 s imaging time for each modality. The 3D RUS-PAT is a powerful tool for high-speed, 3D, dual-contrast imaging of the human body with potential for rapid clinical translation.
Via
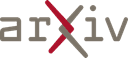
Apr 21, 2025
Abstract:Hip fractures represent a major health concern, particularly among the elderly, often leading decreased mobility and increased mortality. Early and accurate detection of at risk individuals is crucial for effective intervention. In this study, we propose Iterative Cross Graph Matching for Hip Fracture Risk Assessment (ICGM-FRAX), a novel approach for predicting hip fractures using Dual-energy X-ray Absorptiometry (DXA) images. ICGM-FRAX involves iteratively comparing a test (subject) graph with multiple template graphs representing the characteristics of hip fracture subjects to assess the similarity and accurately to predict hip fracture risk. These graphs are obtained as follows. The DXA images are separated into multiple regions of interest (RoIs), such as the femoral head, shaft, and lesser trochanter. Radiomic features are then calculated for each RoI, with the central coordinates used as nodes in a graph. The connectivity between nodes is established according to the Euclidean distance between these coordinates. This process transforms each DXA image into a graph, where each node represents a RoI, and edges derived by the centroids of RoIs capture the spatial relationships between them. If the test graph closely matches a set of template graphs representing subjects with incident hip fractures, it is classified as indicating high hip fracture risk. We evaluated our method using 547 subjects from the UK Biobank dataset, and experimental results show that ICGM-FRAX achieved a sensitivity of 0.9869, demonstrating high accuracy in predicting hip fractures.
* 23 pages, 4 figures
Via
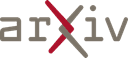
Apr 15, 2025
Abstract:Gaze estimation, which predicts gaze direction, commonly faces the challenge of interference from complex gaze-irrelevant information in face images. In this work, we propose DMAGaze, a novel gaze estimation framework that exploits information from facial images in three aspects: gaze-relevant global features (disentangled from facial image), local eye features (extracted from cropped eye patch), and head pose estimation features, to improve overall performance. Firstly, we design a new continuous mask-based Disentangler to accurately disentangle gaze-relevant and gaze-irrelevant information in facial images by achieving the dual-branch disentanglement goal through separately reconstructing the eye and non-eye regions. Furthermore, we introduce a new cascaded attention module named Multi-Scale Global Local Attention Module (MS-GLAM). Through a customized cascaded attention structure, it effectively focuses on global and local information at multiple scales, further enhancing the information from the Disentangler. Finally, the global gaze-relevant features disentangled by the upper face branch, combined with head pose and local eye features, are passed through the detection head for high-precision gaze estimation. Our proposed DMAGaze has been extensively validated on two mainstream public datasets, achieving state-of-the-art performance.
Via
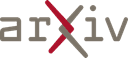
Apr 16, 2025
Abstract:Neural networks often have identifiable computational structures - components of the network which perform an interpretable algorithm or task - but the mechanisms by which these emerge and the best methods for detecting these structures are not well understood. In this paper we investigate the emergence of computational structure in a transformer-like model trained to simulate the physics of a particle system, where the transformer's attention mechanism is used to transfer information between particles. We show that (a) structures emerge in the attention heads of the transformer which learn to detect particle collisions, (b) the emergence of these structures is associated to degenerate geometry in the loss landscape, and (c) the dynamics of this emergence follows a power law. This suggests that these components are governed by a degenerate "effective potential". These results have implications for the convergence time of computational structure within neural networks and suggest that the emergence of computational structure can be detected by studying the dynamics of network components.
* 35 pages
Via
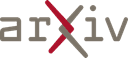
Apr 14, 2025
Abstract:Memes have become a dominant form of communication in social media in recent years. Memes are typically humorous and harmless, however there are also memes that promote hate speech, being in this way harmful to individuals and groups based on their identity. Therefore, detecting hateful content in memes has emerged as a task of critical importance. The need for understanding the complex interactions of images and their embedded text renders the hateful meme detection a challenging multimodal task. In this paper we propose to address the aforementioned task leveraging knowledge encoded in powerful Large Multimodal Models (LMM). Specifically, we propose to exploit LMMs in a two-fold manner. First, by extracting knowledge oriented to the hateful meme detection task in order to build strong meme representations. Specifically, generic semantic descriptions and emotions that the images along with their embedded texts elicit are extracted, which are then used to train a simple classification head for hateful meme detection. Second, by developing a novel hard mining approach introducing directly LMM-encoded knowledge to the training process, providing further improvements. We perform extensive experiments on two datasets that validate the effectiveness of the proposed method, achieving state-of-the-art performance. Our code and trained models are publicly available at: https://github.com/IDT-ITI/LMM-CLIP-meme.
* Accepted for publication, Multimodal Learning and Applications
Workshop (MULA 2025) @ IEEE/CVF CVPR 2025, Nashville, TN, USA, June 2025.
This is the authors' "accepted version"
Via
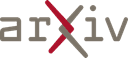
Apr 15, 2025
Abstract:RT-DETRs have shown strong performance across various computer vision tasks but are known to degrade under challenging weather conditions such as fog. In this work, we investigate three novel approaches to enhance RT-DETR robustness in foggy environments: (1) Domain Adaptation via Perceptual Loss, which distills domain-invariant features from a teacher network to a student using perceptual supervision; (2) Weather Adaptive Attention, which augments the attention mechanism with fog-sensitive scaling by introducing an auxiliary foggy image stream; and (3) Weather Fusion Encoder, which integrates a dual-stream encoder architecture that fuses clear and foggy image features via multi-head self and cross-attention. Despite the architectural innovations, none of the proposed methods consistently outperform the baseline RT-DETR. We analyze the limitations and potential causes, offering insights for future research in weather-aware object detection.
Via
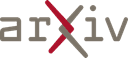
Apr 14, 2025
Abstract:Radio Frequency Interference (RFI) from anthropogenic radio sources poses significant challenges to current and future radio telescopes. Contemporary approaches to detecting RFI treat the task as a semantic segmentation problem on radio telescope spectrograms. Typically, complex heuristic algorithms handle this task of `flagging' in combination with manual labeling (in the most difficult cases). While recent machine-learning approaches have demonstrated high accuracy, they often fail to meet the stringent operational requirements of modern radio observatories. Owing to their inherently time-varying nature, spiking neural networks (SNNs) are a promising alternative method to RFI-detection by utilizing the time-varying nature of the spectrographic source data. In this work, we apply Liquid State Machines (LSMs), a class of spiking neural networks, to RFI-detection. We employ second-order Leaky Integrate-and-Fire (LiF) neurons, marking the first use of this architecture and neuron type for RFI-detection. We test three encoding methods and three increasingly complex readout layers, including a transformer decoder head, providing a hybrid of SNN and ANN techniques. Our methods extend LSMs beyond conventional classification tasks to fine-grained spatio-temporal segmentation. We train LSMs on simulated data derived from the Hyrogen Epoch of Reionization Array (HERA), a known benchmark for RFI-detection. Our model achieves a per-pixel accuracy of 98% and an F1-score of 0.743, demonstrating competitive performance on this highly challenging task. This work expands the sophistication of SNN techniques and architectures applied to RFI-detection, and highlights the effectiveness of LSMs in handling fine-grained, complex, spatio-temporal signal-processing tasks.
* 7 pages, 2 figures, 5 tables, accepted for publication at IJCNN 2025
Via
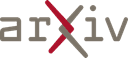