What is Object Detection? Object detection is a computer vision task in which the goal is to detect and locate objects of interest in an image or video. The task involves identifying the position and boundaries of objects in an image, and classifying the objects into different categories. It forms a crucial part of vision recognition, alongside image classification and retrieval.
Papers and Code
Apr 15, 2025
Abstract:Recognizing safety violations in construction environments is critical yet remains underexplored in computer vision. Existing models predominantly rely on 2D object detection, which fails to capture the complexities of real-world violations due to: (i) an oversimplified task formulation treating violation recognition merely as object detection, (ii) inadequate validation under realistic conditions, (iii) absence of standardized baselines, and (iv) limited scalability from the unavailability of synthetic dataset generators for diverse construction scenarios. To address these challenges, we introduce Safe-Construct, the first framework that reformulates violation recognition as a 3D multi-view engagement task, leveraging scene-level worker-object context and 3D spatial understanding. We also propose the Synthetic Indoor Construction Site Generator (SICSG) to create diverse, scalable training data, overcoming data limitations. Safe-Construct achieves a 7.6% improvement over state-of-the-art methods across four violation types. We rigorously evaluate our approach in near-realistic settings, incorporating four violations, four workers, 14 objects, and challenging conditions like occlusions (worker-object, worker-worker) and variable illumination (back-lighting, overexposure, sunlight). By integrating 3D multi-view spatial understanding and synthetic data generation, Safe-Construct sets a new benchmark for scalable and robust safety monitoring in high-risk industries. Project Website: https://Safe-Construct.github.io/Safe-Construct
Via
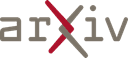
Apr 16, 2025
Abstract:Unmanned Aerial Vehicles (UAVs) are indispensable for infrastructure inspection, surveillance, and related tasks, yet they also introduce critical security challenges. This survey provides a wide-ranging examination of the anti-UAV domain, centering on three core objectives-classification, detection, and tracking-while detailing emerging methodologies such as diffusion-based data synthesis, multi-modal fusion, vision-language modeling, self-supervised learning, and reinforcement learning. We systematically evaluate state-of-the-art solutions across both single-modality and multi-sensor pipelines (spanning RGB, infrared, audio, radar, and RF) and discuss large-scale as well as adversarially oriented benchmarks. Our analysis reveals persistent gaps in real-time performance, stealth detection, and swarm-based scenarios, underscoring pressing needs for robust, adaptive anti-UAV systems. By highlighting open research directions, we aim to foster innovation and guide the development of next-generation defense strategies in an era marked by the extensive use of UAVs.
* Accepted at CVPR Workshop Anti-UAV 2025. 15 pages
Via
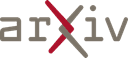
Apr 15, 2025
Abstract:Autonomous systems deployed on edge devices face significant challenges, including resource constraints, real-time processing demands, and adapting to dynamic environments. This work introduces ATLASv2, a novel system that integrates a fine-tuned TinyLLM, real-time object detection, and efficient path planning to enable hierarchical, multi-task navigation and manipulation all on the edge device, Jetson Nano. ATLASv2 dynamically expands its navigable landmarks by detecting and localizing objects in the environment which are saved to its internal knowledge base to be used for future task execution. We evaluate ATLASv2 in real-world environments, including a handcrafted home and office setting constructed with diverse objects and landmarks. Results show that ATLASv2 effectively interprets natural language instructions, decomposes them into low-level actions, and executes tasks with high success rates. By leveraging generative AI in a fully on-board framework, ATLASv2 achieves optimized resource utilization with minimal prompting latency and power consumption, bridging the gap between simulated environments and real-world applications.
Via
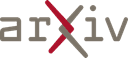
Apr 14, 2025
Abstract:Moving object detection (MOD) in remote sensing is significantly challenged by low resolution, extremely small object sizes, and complex noise interference. Current deep learning-based MOD methods rely on probability density estimation, which restricts flexible information interaction between objects and across temporal frames. To flexibly capture high-order inter-object and temporal relationships, we propose a point-based MOD in remote sensing. Inspired by diffusion models, the network optimization is formulated as a progressive denoising process that iteratively recovers moving object centers from sparse noisy points. Specifically, we sample scattered features from the backbone outputs as atomic units for subsequent processing, while global feature embeddings are aggregated to compensate for the limited coverage of sparse point features. By modeling spatial relative positions and semantic affinities, Spatial Relation Aggregation Attention is designed to enable high-order interactions among point-level features for enhanced object representation. To enhance temporal consistency, the Temporal Propagation and Global Fusion module is designed, which leverages an implicit memory reasoning mechanism for robust cross-frame feature integration. To align with the progressive denoising process, we propose a progressive MinK optimal transport assignment strategy that establishes specialized learning objectives at each denoising level. Additionally, we introduce a missing loss function to counteract the clustering tendency of denoised points around salient objects. Experiments on the RsData remote sensing MOD dataset show that our MOD method based on scattered point denoising can more effectively explore potential relationships between sparse moving objects and improve the detection capability and temporal consistency.
* 9 pages, 7 figures
Via
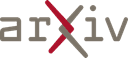
Apr 19, 2025
Abstract:Background: Automated classification of thyroid fine needle aspiration biopsy (FNAB) images faces challenges in limited data, inter-observer variability, and computational cost. Efficient, interpretable models are crucial for clinical support. Objective: To develop and externally validate a deep learning system for the multi-class classification of thyroid FNAB images into three key categories that directly guide post-biopsy treatment decisions in Vietnam: benign (B2), suspicious for malignancy (B5), and malignant (B6), while achieving high diagnostic accuracy with low computational overhead. Methods: Our framework features: (1) YOLOv10-based cell cluster detection for informative sub-region extraction and noise reduction; (2) a curriculum learning-inspired protocol sequencing localized crops to full images for multi-scale feature capture; (3) adaptive lightweight EfficientNetB0 (4 millions parameters) selection balancing performance and efficiency; and (4) a Transformer-inspired module for multi-scale, multi-region analysis. External validation used 1,015 independent FNAB images. Results: ThyroidEffi Basic achieved a macro F1 of 89.19\% and AUCs of 0.98 (B2), 0.95 (B5), and 0.96 (B6) on the internal test set. External validation yielded AUCs of 0.9495 (B2), 0.7436 (B5), and 0.8396 (B6). ThyroidEffi Premium improved macro F1 to 89.77\%. Grad-CAM highlighted key diagnostic regions, confirming interpretability. The system processed 1000 cases in 30 seconds, demonstrating feasibility on widely accessible hardware like a 12-core CPU. Conclusions: This work demonstrates that high-accuracy, interpretable thyroid FNAB image classification is achievable with minimal computational demands.
Via
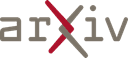
Apr 15, 2025
Abstract:The World Health Organization suggests that road traffic crashes cost approximately 518 billion dollars globally each year, which accounts for 3% of the gross domestic product for most countries. Most fatal road accidents in urban areas involve Vulnerable Road Users (VRUs). Smart cities environments present innovative approaches to combat accidents involving cutting-edge technologies, that include advanced sensors, extensive datasets, Machine Learning (ML) models, communication systems, and edge computing. This paper proposes a strategy and an implementation of a system for road monitoring and safety for smart cities, based on Computer Vision (CV) and edge computing. Promising results were obtained by implementing vision algorithms and tracking using surveillance cameras, that are part of a Smart City testbed, the Aveiro Tech City Living Lab (ATCLL). The algorithm accurately detects and tracks cars, pedestrians, and bicycles, while predicting the road state, the distance between moving objects, and inferring on collision events to prevent collisions, in near real-time.
* 30th ITS World Congress, Dubai, UAE, 16-20 September 2024
Via
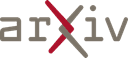
Apr 16, 2025
Abstract:Visual relation detection (VRD) aims to identify relationships (or interactions) between object pairs in an image. Although recent VRD models have achieved impressive performance, they are all restricted to pre-defined relation categories, while failing to consider the semantic ambiguity characteristic of visual relations. Unlike objects, the appearance of visual relations is always subtle and can be described by multiple predicate words from different perspectives, e.g., ``ride'' can be depicted as ``race'' and ``sit on'', from the sports and spatial position views, respectively. To this end, we propose to model visual relations as continuous embeddings, and design diffusion models to achieve generalized VRD in a conditional generative manner, termed Diff-VRD. We model the diffusion process in a latent space and generate all possible relations in the image as an embedding sequence. During the generation, the visual and text embeddings of subject-object pairs serve as conditional signals and are injected via cross-attention. After the generation, we design a subsequent matching stage to assign the relation words to subject-object pairs by considering their semantic similarities. Benefiting from the diffusion-based generative process, our Diff-VRD is able to generate visual relations beyond the pre-defined category labels of datasets. To properly evaluate this generalized VRD task, we introduce two evaluation metrics, i.e., text-to-image retrieval and SPICE PR Curve inspired by image captioning. Extensive experiments in both human-object interaction (HOI) detection and scene graph generation (SGG) benchmarks attest to the superiority and effectiveness of Diff-VRD.
* Under review at IEEE TCSVT. The Appendix is provided additionally
Via
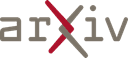
Apr 15, 2025
Abstract:Wood defect detection is critical for ensuring quality control in the wood processing industry. However, current industrial applications face two major challenges: traditional methods are costly, subjective, and labor-intensive, while mainstream deep learning models often struggle to balance detection accuracy and computational efficiency for edge deployment. To address these issues, this study proposes CFIS-YOLO, a lightweight object detection model optimized for edge devices. The model introduces an enhanced C2f structure, a dynamic feature recombination module, and a novel loss function that incorporates auxiliary bounding boxes and angular constraints. These innovations improve multi-scale feature fusion and small object localization while significantly reducing computational overhead. Evaluated on a public wood defect dataset, CFIS-YOLO achieves a mean Average Precision (mAP@0.5) of 77.5\%, outperforming the baseline YOLOv10s by 4 percentage points. On SOPHON BM1684X edge devices, CFIS-YOLO delivers 135 FPS, reduces power consumption to 17.3\% of the original implementation, and incurs only a 0.5 percentage point drop in mAP. These results demonstrate that CFIS-YOLO is a practical and effective solution for real-world wood defect detection in resource-constrained environments.
* 10 pages, 11 figures
Via
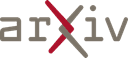
Apr 15, 2025
Abstract:Reliable traffic data are essential for understanding urban mobility and developing effective traffic management strategies. This study introduces the DRone-derived Intelligence For Traffic analysis (DRIFT) dataset, a large-scale urban traffic dataset collected systematically from synchronized drone videos at approximately 250 meters altitude, covering nine interconnected intersections in Daejeon, South Korea. DRIFT provides high-resolution vehicle trajectories that include directional information, processed through video synchronization and orthomap alignment, resulting in a comprehensive dataset of 81,699 vehicle trajectories. Through our DRIFT dataset, researchers can simultaneously analyze traffic at multiple scales - from individual vehicle maneuvers like lane-changes and safety metrics such as time-to-collision to aggregate network flow dynamics across interconnected urban intersections. The DRIFT dataset is structured to enable immediate use without additional preprocessing, complemented by open-source models for object detection and trajectory extraction, as well as associated analytical tools. DRIFT is expected to significantly contribute to academic research and practical applications, such as traffic flow analysis and simulation studies. The dataset and related resources are publicly accessible at https://github.com/AIxMobility/The-DRIFT.
* 30 pages, 15 figures
Via
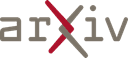
Apr 14, 2025
Abstract:Compared with the generic scenes, crowded scenes contain highly-overlapped instances, which result in: 1) more ambiguous anchors during training of object detectors, and 2) more predictions are likely to be mistakenly suppressed in post-processing during inference. To address these problems, we propose two new strategies, density-guided anchors (DGA) and density-guided NMS (DG-NMS), which uses object density maps to jointly compute optimal anchor assignments and reweighing, as well as an adaptive NMS. Concretely, based on an unbalanced optimal transport (UOT) problem, the density owned by each ground-truth object is transported to each anchor position at a minimal transport cost. And density on anchors comprises an instance-specific density distribution, from which DGA decodes the optimal anchor assignment and re-weighting strategy. Meanwhile, DG-NMS utilizes the predicted density map to adaptively adjust the NMS threshold to reduce mistaken suppressions. In the UOT, a novel overlap-aware transport cost is specifically designed for ambiguous anchors caused by overlapped neighboring objects. Extensive experiments on the challenging CrowdHuman dataset with Citypersons dataset demonstrate that our proposed density-guided detector is effective and robust to crowdedness. The code and pre-trained models will be made available later.
Via
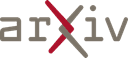