Topic:Music Source Separation
What is Music Source Separation? Music source separation is the process of separating individual sound sources from a mixed audio signal.
Papers and Code
Apr 07, 2025
Abstract:Artistic style has been studied for centuries, and recent advances in machine learning create new possibilities for understanding it computationally. However, ensuring that machine-learning models produce insights aligned with the interests of practitioners and critics remains a significant challenge. Here, we focus on musical style, which benefits from a rich theoretical and mathematical analysis tradition. We train a variety of supervised-learning models to identify 20 iconic jazz musicians across a carefully curated dataset of 84 hours of recordings, and interpret their decision-making processes. Our models include a novel multi-input architecture that enables four musical domains (melody, harmony, rhythm, and dynamics) to be analysed separately. These models enable us to address fundamental questions in music theory and also advance the state-of-the-art in music performer identification (94% accuracy across 20 classes). We release open-source implementations of our models and an accompanying web application for exploring musical styles.
* Paper: 40 pages, 11 figures, 1 table. Supplementary material: 33
pages, 48 figures, 6 tables
Via
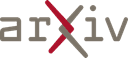
Apr 04, 2025
Abstract:Robot audition, encompassing Sound Source Localization (SSL), Sound Source Separation (SSS), and Automatic Speech Recognition (ASR), enables robots and smart devices to acquire auditory capabilities similar to human hearing. Despite their wide applicability, processing multi-channel audio signals from microphone arrays in SSL involves computationally intensive matrix operations, which can hinder efficient deployment on Central Processing Units (CPUs), particularly in embedded systems with limited CPU resources. This paper introduces a GPU-based implementation of SSL for robot audition, utilizing the Generalized Singular Value Decomposition-based Multiple Signal Classification (GSVD-MUSIC), a noise-robust algorithm, within the HARK platform, an open-source software suite. For a 60-channel microphone array, the proposed implementation achieves significant performance improvements. On the Jetson AGX Orin, an embedded device powered by an NVIDIA GPU and ARM Cortex-A78AE v8.2 64-bit CPUs, we observe speedups of 4645.1x for GSVD calculations and 8.8x for the SSL module, while speedups of 2223.4x for GSVD calculation and 8.95x for the entire SSL module on a server configured with an NVIDIA A100 GPU and AMD EPYC 7352 CPUs, making real-time processing feasible for large-scale microphone arrays and providing ample capacity for real-time processing of potential subsequent machine learning or deep learning tasks.
* 6 pages, 2 figures
Via
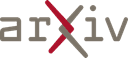
Mar 31, 2025
Abstract:We propose Universal target audio Separation (UniSep), addressing the separation task on arbitrary mixtures of different types of audio. Distinguished from previous studies, UniSep is performed on unlimited source domains and unlimited source numbers. We formulate the separation task as a sequence-to-sequence problem, and a large language model (LLM) is used to model the audio sequence in the discrete latent space, leveraging the power of LLM in handling complex mixture audios with large-scale data. Moreover, a novel pre-training strategy is proposed to utilize audio-only data, which reduces the efforts of large-scale data simulation and enhances the ability of LLMs to understand the consistency and correlation of information within audio sequences. We also demonstrate the effectiveness of scaling datasets in an audio separation task: we use large-scale data (36.5k hours), including speech, music, and sound, to train a universal target audio separation model that is not limited to a specific domain. Experiments show that UniSep achieves competitive subjective and objective evaluation results compared with single-task models.
* Accepted by ICME 2025
Via
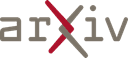
Mar 10, 2025
Abstract:Music source separation is the task of separating a mixture of instruments into constituent tracks. Music source separation models are typically trained using only audio data, although additional information can be used to improve the model's separation capability. In this paper, we propose two ways of using musical scores to aid music source separation: a score-informed model where the score is concatenated with the magnitude spectrogram of the audio mixture as the input of the model, and a model where we use only the score to calculate the separation mask. We train our models on synthetic data in the SynthSOD dataset and evaluate our methods on the URMP and Aalto anechoic orchestra datasets, comprised of real recordings. The score-informed model improves separation results compared to a baseline approach, but struggles to generalize from synthetic to real data, whereas the score-only model shows a clear improvement in synthetic-to-real generalization.
* 5 pages, 2 figures, submitted to Eusipco2025
Via
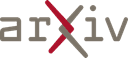
Mar 06, 2025
Abstract:Musical source separation (MSS) has recently seen a big breakthrough in separating instruments from a mixture in the context of Western music, but research on non-Western instruments is still limited due to a lack of data. In this demo, we use an existing dataset of Brazilian sama percussion to create artificial mixtures for training a U-Net model to separate the surdo drum, a traditional instrument in samba. Despite limited training data, the model effectively isolates the surdo, given the drum's repetitive patterns and its characteristic low-pitched timbre. These results suggest that MSS systems can be successfully harnessed to work in more culturally-inclusive scenarios without the need of collecting extensive amounts of data.
* 2 pages + references, 1 figure, 1 table, Extended Abstracts for the
Late-Breaking Demo Session of the 25th International Society for Music
Information Retrieval Conference
Via
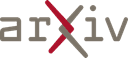
Jan 27, 2025
Abstract:Music source separation is an audio-to-audio retrieval task of extracting one or more constituent components, or composites thereof, from a musical audio mixture. Each of these constituent components is often referred to as a "stem" in literature. Historically, music source separation has been dominated by a stem-based paradigm, leading to most state-of-the-art systems being either a collection of single-stem extraction models, or a tightly coupled system with a fixed, difficult-to-modify, set of supported stems. Combined with the limited data availability, advances in music source separation have thus been mostly limited to the "VDBO" set of stems: \textit{vocals}, \textit{drum}, \textit{bass}, and the catch-all \textit{others}. Recent work in music source separation has begun to challenge the fixed-stem paradigm, moving towards models able to extract any musical sound as long as this target type of sound could be specified to the model as an additional query input. We generalize this idea to a \textit{query-by-region} source separation system, specifying the target based on the query regardless of how many sound sources or which sound classes are contained within it. To do so, we propose the use of hyperellipsoidal regions as queries to allow for an intuitive yet easily parametrizable approach to specifying both the target (location) as well as its spread. Evaluation of the proposed system on the MoisesDB dataset demonstrated state-of-the-art performance of the proposed system both in terms of signal-to-noise ratios and retrieval metrics.
* Submitted to the 2025 International Joint Conference on Artificial
Intelligence
Via
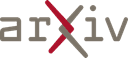
Feb 10, 2025
Abstract:Sampling, the practice of reusing recorded music or sounds from another source in a new work, is common in popular music genres like hip-hop and rap. Numerous services have emerged that allow users to identify connections between samples and the songs that incorporate them, with the goal of enhancing music discovery. Designing a system that can perform the same task automatically is challenging, as samples are commonly altered with audio effects like pitch- and time-stretching and may only be seconds long. Progress on this task has been minimal and is further blocked by the limited availability of training data. Here, we show that a convolutional neural network trained on an artificial dataset can identify real-world samples in commercial hip-hop music. We extract vocal, harmonic, and percussive elements from several databases of non-commercial music recordings using audio source separation, and train the model to fingerprint a subset of these elements in transformed versions of the original audio. We optimize the model using a joint classification and metric learning loss and show that it achieves 13% greater precision on real-world instances of sampling than a fingerprinting system using acoustic landmarks, and that it can recognize samples that have been both pitch shifted and time stretched. We also show that, for half of the commercial music recordings we tested, our model is capable of locating the position of a sample to within five seconds.
* 17 pages, 6 figures
Via
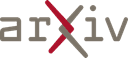
Feb 18, 2025
Abstract:Text-to-song generation, the task of creating vocals and accompaniment from textual inputs, poses significant challenges due to domain complexity and data scarcity. Existing approaches often employ multi-stage generation procedures, resulting in cumbersome training and inference pipelines. In this paper, we propose SongGen, a fully open-source, single-stage auto-regressive transformer designed for controllable song generation. The proposed model facilitates fine-grained control over diverse musical attributes, including lyrics and textual descriptions of instrumentation, genre, mood, and timbre, while also offering an optional three-second reference clip for voice cloning. Within a unified auto-regressive framework, SongGen supports two output modes: mixed mode, which generates a mixture of vocals and accompaniment directly, and dual-track mode, which synthesizes them separately for greater flexibility in downstream applications. We explore diverse token pattern strategies for each mode, leading to notable improvements and valuable insights. Furthermore, we design an automated data preprocessing pipeline with effective quality control. To foster community engagement and future research, we will release our model weights, training code, annotated data, and preprocessing pipeline. The generated samples are showcased on our project page at https://liuzh-19.github.io/SongGen/ , and the code will be available at https://github.com/LiuZH-19/SongGen .
Via
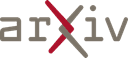
Jan 07, 2025
Abstract:Music source separation and pitch estimation are two vital tasks in music information retrieval. Typically, the input of pitch estimation is obtained from the output of music source separation. Therefore, existing methods have tried to perform these two tasks simultaneously, so as to leverage the mutually beneficial relationship between both tasks. However, these methods still face two critical challenges that limit the improvement of both tasks: the lack of labeled data and joint learning optimization. To address these challenges, we propose a Model-Agnostic Joint Learning (MAJL) framework for both tasks. MAJL is a generic framework and can use variant models for each task. It includes a two-stage training method and a dynamic weighting method named Dynamic Weights on Hard Samples (DWHS), which addresses the lack of labeled data and joint learning optimization, respectively. Experimental results on public music datasets show that MAJL outperforms state-of-the-art methods on both tasks, with significant improvements of 0.92 in Signal-to-Distortion Ratio (SDR) for music source separation and 2.71% in Raw Pitch Accuracy (RPA) for pitch estimation. Furthermore, comprehensive studies not only validate the effectiveness of each component of MAJL, but also indicate the great generality of MAJL in adapting to different model architectures.
Via
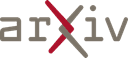
Jan 12, 2025
Abstract:Music source separation demixes a piece of music into its individual sound sources (vocals, percussion, melodic instruments, etc.), a task with no simple mathematical solution. It requires deep learning methods involving training on large datasets of isolated music stems. The most commonly available datasets are made from commercial Western music, limiting the models' applications to non-Western genres like Carnatic music. Carnatic music is a live tradition, with the available multi-track recordings containing overlapping sounds and bleeds between the sources. This poses a challenge to commercially available source separation models like Spleeter and Hybrid Demucs. In this work, we introduce 'Sanidha', the first open-source novel dataset for Carnatic music, offering studio-quality, multi-track recordings with minimal to no overlap or bleed. Along with the audio files, we provide high-definition videos of the artists' performances. Additionally, we fine-tuned Spleeter, one of the most commonly used source separation models, on our dataset and observed improved SDR performance compared to fine-tuning on a pre-existing Carnatic multi-track dataset. The outputs of the fine-tuned model with 'Sanidha' are evaluated through a listening study.
* Accepted to the 25th International Society for Music Information
Retrieval Conference (ISMIR 2024)
Via
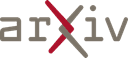