What is Generative AI? Generative AI or generative artificial intelligence refers to a type of AI that can create various types of content including text, audio, music, images, videos, and code. This is powered by large models called foundation models that are trained on massive datasets to perform out-of-the-box tasks including classification, summarization, video and audio comprehension, prediction, Q&A, and more.
Papers and Code
Nov 15, 2024
Abstract:As the boundaries of human computer interaction expand, Generative AI emerges as a key driver in reshaping user interfaces, introducing new possibilities for personalized, multimodal and cross-platform interactions. This integration reflects a growing demand for more adaptive and intuitive user interfaces that can accommodate diverse input types such as text, voice and video, and deliver seamless experiences across devices. This paper explores the integration of generative AI in modern user interfaces, examining historical developments and focusing on multimodal interaction, cross-platform adaptability and dynamic personalization. A central theme is the interface dilemma, which addresses the challenge of designing effective interactions for multimodal large language models, assessing the trade-offs between graphical, voice-based and immersive interfaces. The paper further evaluates lightweight frameworks tailored for mobile platforms, spotlighting the role of mobile hardware in enabling scalable multimodal AI. Technical and ethical challenges, including context retention, privacy concerns and balancing cloud and on-device processing are thoroughly examined. Finally, the paper outlines future directions such as emotionally adaptive interfaces, predictive AI driven user interfaces and real-time collaborative systems, underscoring generative AI's potential to redefine adaptive user-centric interfaces across platforms.
* 13 pages, 4 figures
Via
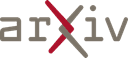
Nov 15, 2024
Abstract:This white paper is the output of a multidisciplinary workshop in Nairobi (Nov 2023). Led by a cross-organisational team including Microsoft Research, NEPAD, Lelapa AI, and University of Oxford. The workshop brought together diverse thought-leaders from various sectors and backgrounds to discuss the implications of Generative AI for the future of work in Africa. Discussions centred around four key themes: Macroeconomic Impacts; Jobs, Skills and Labour Markets; Workers' Perspectives and Africa-Centris AI Platforms. The white paper provides an overview of the current state and trends of generative AI and its applications in different domains, as well as the challenges and risks associated with its adoption and regulation. It represents a diverse set of perspectives to create a set of insights and recommendations which aim to encourage debate and collaborative action towards creating a dignified future of work for everyone across Africa.
Via
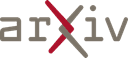
Nov 15, 2024
Abstract:This paper explores how Large Language Models (LLMs) can automate consensus-seeking in supply chain management (SCM), where frequent decisions on problems such as inventory levels and delivery times require coordination among companies. Traditional SCM relies on human consensus in decision-making to avoid emergent problems like the bullwhip effect. Some routine consensus processes, especially those that are time-intensive and costly, can be automated. Existing solutions for automated coordination have faced challenges due to high entry barriers locking out SMEs, limited capabilities, and limited adaptability in complex scenarios. However, recent advances in Generative AI, particularly LLMs, show promise in overcoming these barriers. LLMs, trained on vast datasets can negotiate, reason, and plan, facilitating near-human-level consensus at scale with minimal entry barriers. In this work, we identify key limitations in existing approaches and propose autonomous LLM agents to address these gaps. We introduce a series of novel, supply chain-specific consensus-seeking frameworks tailored for LLM agents and validate the effectiveness of our approach through a case study in inventory management. To accelerate progress within the SCM community, we open-source our code, providing a foundation for further advancements in LLM-powered autonomous supply chain solutions.
Via
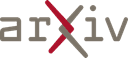
Nov 14, 2024
Abstract:The demands for accurate and representative generative AI systems means there is an increased demand on participatory evaluation structures. While these participatory structures are paramount to to ensure non-dominant values, knowledge and material culture are also reflected in AI models and the media they generate, we argue that dominant structures of community participation in AI development and evaluation are not explicit enough about the benefits and harms that members of socially marginalized groups may experience as a result of their participation. Without explicit interrogation of these benefits by AI developers, as a community we may remain blind to the immensity of systemic change that is needed as well. To support this provocation, we present a speculative case study, developed from our own collective experiences as AI researchers. We use this speculative context to itemize the barriers that need to be overcome in order for the proposed benefits to marginalized communities to be realized, and harms mitigated.
* 3 pages, 1 figure. Published as a Short Paper in the NeurIPS 2024
Workshop on Evaluating Evaluations: Examining Best Practices for Measuring
Broader Impacts of Generative AI
Via
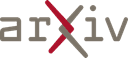
Nov 15, 2024
Abstract:Digital media platforms (e.g., social media, science blogs) offer opportunities to communicate scientific content to general audiences at scale. However, these audiences vary in their scientific expertise, literacy levels, and personal backgrounds, making effective science communication challenging. To address this challenge, we designed TranSlider, an AI-powered tool that generates personalized translations of scientific text based on individual user profiles (e.g., hobbies, location, and education). Our tool features an interactive slider that allows users to steer the degree of personalization from 0 (weakly relatable) to 100 (strongly relatable), leveraging LLMs to generate the translations with given degrees. Through an exploratory study with 15 participants, we investigated both the utility of these AI-personalized translations and how interactive reading features influenced users' understanding and reading experiences. We found that participants who preferred higher degrees of personalization appreciated the relatable and contextual translations, while those who preferred lower degrees valued concise translations with subtle contextualization. Furthermore, participants reported the compounding effect of multiple translations on their understanding of scientific content. Given these findings, we discuss several implications of AI-personalized translation tools in facilitating communication in collaborative contexts.
* 23 pages, 5 figures, 1 table
Via
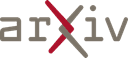
Nov 14, 2024
Abstract:ABCI 3.0 is the latest version of the ABCI, a large-scale open AI infrastructure that AIST has been operating since August 2018 and will be fully operational in January 2025. ABCI 3.0 consists of computing servers equipped with 6128 of the NVIDIA H200 GPUs and an all-flash storage system. Its peak performance is 6.22 exaflops in half precision and 3.0 exaflops in single precision, which is 7 to 13 times faster than the previous system, ABCI 2.0. It also more than doubles both storage capacity and theoretical read/write performance. ABCI 3.0 is expected to accelerate research and development, evaluation, and workforce development of cutting-edge AI technologies, with a particular focus on generative AI.
* 4 pages, 2 figures
Via
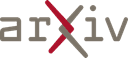
Nov 14, 2024
Abstract:We present the MIDInfinite, a web application capable of generating symbolic music using a large-scale generative AI model locally on commodity hardware. Creating this demo involved porting the Anticipatory Music Transformer, a large language model (LLM) pre-trained on the Lakh MIDI dataset, to the Machine Learning Compilation (MLC) framework. Once the model is ported, MLC facilitates inference on a variety of runtimes including C++, mobile, and the browser. We envision that MLC has the potential to bridge the gap between the landscape of increasingly capable music AI models and technology more familiar to music software developers. As a proof of concept, we build a web application that allows users to generate endless streams of multi-instrumental MIDI in the browser, either from scratch or conditioned on a prompt. On commodity hardware (an M3 Macbook Pro), our demo can generate 51 notes per second, which is faster than real-time playback for 72.9% of generations, and increases to 86.3% with 2 seconds of upfront buffering.
* 2 pages
Via
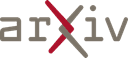
Nov 15, 2024
Abstract:Electroencephalography (EEG) plays a crucial role in the diagnosis of various neurological disorders. However, small hospitals and clinics often lack advanced EEG signal analysis systems and are prone to misinterpretation in manual EEG reading. This study proposes an innovative hybrid artificial intelligence (AI) system for automatic interpretation of EEG background activity and report generation. The system combines deep learning models for posterior dominant rhythm (PDR) prediction, unsupervised artifact removal, and expert-designed algorithms for abnormality detection. For PDR prediction, 1530 labeled EEGs were used, and the best ensemble model achieved a mean absolute error (MAE) of 0.237, a root mean square error (RMSE) of 0.359, an accuracy of 91.8% within a 0.6Hz error, and an accuracy of 99% within a 1.2Hz error. The AI system significantly outperformed neurologists in detecting generalized background slowing (p = 0.02; F1: AI 0.93, neurologists 0.82) and demonstrated improved focal abnormality detection, although not statistically significant (p = 0.79; F1: AI 0.71, neurologists 0.55). Validation on both an internal dataset and the Temple University Abnormal EEG Corpus showed consistent performance (F1: 0.884 and 0.835, respectively; p = 0.66), demonstrating generalizability. The use of large language models (LLMs) for report generation demonstrated 100% accuracy, verified by three other independent LLMs. This hybrid AI system provides an easily scalable and accurate solution for EEG interpretation in resource-limited settings, assisting neurologists in improving diagnostic accuracy and reducing misdiagnosis rates.
* IEEE Journal of Biomedical and Health Informatics (2024)
* Example code available at https://github.com/tcs211/AI_EEEG_REPORT
Via
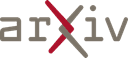
Nov 15, 2024
Abstract:In 2020, we introduced a deep reinforcement learning method capable of generating superhuman chip layouts, which we then published in Nature and open-sourced on GitHub. AlphaChip has inspired an explosion of work on AI for chip design, and has been deployed in state-of-the-art chips across Alphabet and extended by external chipmakers. Even so, a non-peer-reviewed invited paper at ISPD 2023 questioned its performance claims, despite failing to run our method as described in Nature. For example, it did not pre-train the RL method (removing its ability to learn from prior experience), used substantially fewer compute resources (20x fewer RL experience collectors and half as many GPUs), did not train to convergence (standard practice in machine learning), and evaluated on test cases that are not representative of modern chips. Recently, Igor Markov published a meta-analysis of three papers: our peer-reviewed Nature paper, the non-peer-reviewed ISPD paper, and Markov's own unpublished paper (though he does not disclose that he co-authored it). Although AlphaChip has already achieved widespread adoption and impact, we publish this response to ensure that no one is wrongly discouraged from innovating in this impactful area.
Via
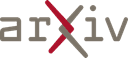
Nov 13, 2024
Abstract:With the rapid advancement of artificial intelligence (AI), generative AI (GenAI) has emerged as a transformative tool, enabling customized and personalized AI-generated content (AIGC) services. However, GenAI models with billions of parameters require substantial memory capacity and computational power for deployment and execution, presenting significant challenges to resource-limited edge networks. In this paper, we address the joint model caching and resource allocation problem in GenAI-enabled wireless edge networks. Our objective is to balance the trade-off between delivering high-quality AIGC and minimizing the delay in AIGC service provisioning. To tackle this problem, we employ a deep deterministic policy gradient (DDPG)-based reinforcement learning approach, capable of efficiently determining optimal model caching and resource allocation decisions for AIGC services in response to user mobility and time-varying channel conditions. Numerical results demonstrate that DDPG achieves a higher model hit ratio and provides superior-quality, lower-latency AIGC services compared to other benchmark solutions.
* conference paper with 6 pages and 5 figures. arXiv admin note: text
overlap with arXiv:2411.01458
Via
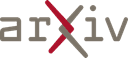