What is Object Detection? Object detection is a computer vision task in which the goal is to detect and locate objects of interest in an image or video. The task involves identifying the position and boundaries of objects in an image, and classifying the objects into different categories. It forms a crucial part of vision recognition, alongside image classification and retrieval.
Papers and Code
Jun 24, 2025
Abstract:Matrix seriation, the problem of permuting the rows and columns of a matrix to uncover latent structure, is a fundamental technique in data science, particularly in the visualization and analysis of relational data. Applications span clustering, anomaly detection, and beyond. In this work, we present a unified framework grounded in mathematical optimization to address matrix seriation from a rigorous, model-based perspective. Our approach leverages combinatorial and mixed-integer optimization to represent seriation objectives and constraints with high fidelity, bridging the gap between traditional heuristic methods and exact solution techniques. We introduce new mathematical programming models for neighborhood-based stress criteria, including nonlinear formulations and their linearized counterparts. For structured settings such as Moore and von Neumann neighborhoods, we develop a novel Hamiltonian path-based reformulation that enables effective control over spatial arrangement and interpretability in the reordered matrix. To assess the practical impact of our models, we carry out an extensive set of experiments on synthetic and real-world datasets, as well as on a newly curated benchmark based on a coauthorship network from the matrix seriation literature. Our results show that these optimization-based formulations not only enhance solution quality and interpretability but also provide a versatile foundation for extending matrix seriation to new domains in data science.
* 30 pages, 20 figures, 8 tables. Codes and datasets available at:
https://github.com/vblancoOR/seriation_mathopt
Via
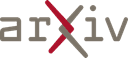
Jun 23, 2025
Abstract:Handling delicate and fragile objects remains a major challenge for robotic manipulation, especially for rigid parallel grippers. While the simplicity and versatility of parallel grippers have led to widespread adoption, these grippers are limited by their heavy reliance on visual feedback. Tactile sensing and soft robotics can add responsiveness and compliance. However, existing methods typically involve high integration complexity or suffer from slow response times. In this work, we introduce FORTE, a tactile sensing system embedded in compliant gripper fingers. FORTE uses 3D-printed fin-ray grippers with internal air channels to provide low-latency force and slip feedback. FORTE applies just enough force to grasp objects without damaging them, while remaining easy to fabricate and integrate. We find that FORTE can accurately estimate grasping forces from 0-8 N with an average error of 0.2 N, and detect slip events within 100 ms of occurring. We demonstrate FORTE's ability to grasp a wide range of slippery, fragile, and deformable objects. In particular, FORTE grasps fragile objects like raspberries and potato chips with a 98.6% success rate, and achieves 93% accuracy in detecting slip events. These results highlight FORTE's potential as a robust and practical solution for enabling delicate robotic manipulation. Project page: https://merge-lab.github.io/FORTE
Via
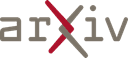
Jun 24, 2025
Abstract:Existing micro aerial vehicle (MAV) detection methods mainly rely on the target's appearance features in RGB images, whose diversity makes it difficult to achieve generalized MAV detection. We notice that different types of MAVs share the same distinctive features in event streams due to their high-speed rotating propellers, which are hard to see in RGB images. This paper studies how to detect different types of MAVs from an event camera by fully exploiting the features of propellers in the original event stream. The proposed method consists of three modules to extract the salient and spatio-temporal features of the propellers while filtering out noise from background objects and camera motion. Since there are no existing event-based MAV datasets, we introduce a novel MAV dataset for the community. This is the first event-based MAV dataset comprising multiple scenarios and different types of MAVs. Without training, our method significantly outperforms state-of-the-art methods and can deal with challenging scenarios, achieving a precision rate of 83.0\% (+30.3\%) and a recall rate of 81.5\% (+36.4\%) on the proposed testing dataset. The dataset and code are available at: https://github.com/WindyLab/EvDetMAV.
* 8 pages, 7 figures. This paper is accepted by IEEE Robotics and
Automation Letters
Via
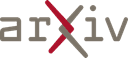
Jun 24, 2025
Abstract:The increasing miniaturization of Unmanned Aerial Vehicles (UAVs) has expanded their deployment potential to indoor and hard-to-reach areas. However, this trend introduces distinct challenges, particularly in terms of flight dynamics and power consumption, which limit the UAVs' autonomy and mission capabilities. This paper presents a novel approach to overcoming these limitations by integrating Neural 3D Reconstruction (N3DR) with small UAV systems for fine-grained 3-Dimensional (3D) digital reconstruction of small static objects. Specifically, we design, implement, and evaluate an N3DR-based pipeline that leverages advanced models, i.e., Instant-ngp, Nerfacto, and Splatfacto, to improve the quality of 3D reconstructions using images of the object captured by a fleet of small UAVs. We assess the performance of the considered models using various imagery and pointcloud metrics, comparing them against the baseline Structure from Motion (SfM) algorithm. The experimental results demonstrate that the N3DR-enhanced pipeline significantly improves reconstruction quality, making it feasible for small UAVs to support high-precision 3D mapping and anomaly detection in constrained environments. In more general terms, our results highlight the potential of N3DR in advancing the capabilities of miniaturized UAV systems.
* 6 pages, 7 figures, 2 tables, accepted at IEEE International
Symposium on Personal, Indoor and Mobile Radio Communications 2025
Via
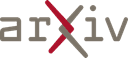
Jun 23, 2025
Abstract:Automated detection of small and rare wildlife in aerial imagery is crucial for effective conservation, yet remains a significant technical challenge. Prairie dogs exemplify this issue: their ecological importance as keystone species contrasts sharply with their elusive presence--marked by small size, sparse distribution, and subtle visual features--which undermines existing detection approaches. To address these challenges, we propose RareSpot, a robust detection framework integrating multi-scale consistency learning and context-aware augmentation. Our multi-scale consistency approach leverages structured alignment across feature pyramids, enhancing fine-grained object representation and mitigating scale-related feature loss. Complementarily, context-aware augmentation strategically synthesizes challenging training instances by embedding difficult-to-detect samples into realistic environmental contexts, significantly boosting model precision and recall. Evaluated on an expert-annotated prairie dog drone imagery benchmark, our method achieves state-of-the-art performance, improving detection accuracy by over 35% compared to baseline methods. Importantly, it generalizes effectively across additional wildlife datasets, demonstrating broad applicability. The RareSpot benchmark and approach not only support critical ecological monitoring but also establish a new foundation for detecting small, rare species in complex aerial scenes.
* Accepted to the CVPR 2025 Workshop on Computer Vision for Animal
Behavior Tracking and Modeling (CV4Animals)
Via
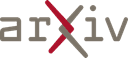
Jun 18, 2025
Abstract:Multispectral object detection, which integrates information from multiple bands, can enhance detection accuracy and environmental adaptability, holding great application potential across various fields. Although existing methods have made progress in cross-modal interaction, low-light conditions, and model lightweight, there are still challenges like the lack of a unified single-stage framework, difficulty in balancing performance and fusion strategy, and unreasonable modality weight allocation. To address these, based on the YOLOv11 framework, we present YOLOv11-RGBT, a new comprehensive multimodal object detection framework. We designed six multispectral fusion modes and successfully applied them to models from YOLOv3 to YOLOv12 and RT-DETR. After reevaluating the importance of the two modalities, we proposed a P3 mid-fusion strategy and multispectral controllable fine-tuning (MCF) strategy for multispectral models. These improvements optimize feature fusion, reduce redundancy and mismatches, and boost overall model performance. Experiments show our framework excels on three major open-source multispectral object detection datasets, like LLVIP and FLIR. Particularly, the multispectral controllable fine-tuning strategy significantly enhanced model adaptability and robustness. On the FLIR dataset, it consistently improved YOLOv11 models' mAP by 3.41%-5.65%, reaching a maximum of 47.61%, verifying the framework and strategies' effectiveness. The code is available at: https://github.com/wandahangFY/YOLOv11-RGBT.
* 29 pages, 8 figures . The errors in the first version have been
corrected, and no new version will be submitted in the near future. The next
version will include more experiments
Via
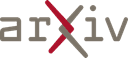
Jun 18, 2025
Abstract:Open-vocabulary 3D object detection has gained significant interest due to its critical applications in autonomous driving and embodied AI. Existing detection methods, whether offline or online, typically rely on dense point cloud reconstruction, which imposes substantial computational overhead and memory constraints, hindering real-time deployment in downstream tasks. To address this, we propose a novel reconstruction-free online framework tailored for memory-efficient and real-time 3D detection. Specifically, given streaming posed RGB-D video input, we leverage Cubify Anything as a pre-trained visual foundation model (VFM) for single-view 3D object detection by bounding boxes, coupled with CLIP to capture open-vocabulary semantics of detected objects. To fuse all detected bounding boxes across different views into a unified one, we employ an association module for correspondences of multi-views and an optimization module to fuse the 3D bounding boxes of the same instance predicted in multi-views. The association module utilizes 3D Non-Maximum Suppression (NMS) and a box correspondence matching module, while the optimization module uses an IoU-guided efficient random optimization technique based on particle filtering to enforce multi-view consistency of the 3D bounding boxes while minimizing computational complexity. Extensive experiments on ScanNetV2 and CA-1M datasets demonstrate that our method achieves state-of-the-art performance among online methods. Benefiting from this novel reconstruction-free paradigm for 3D object detection, our method exhibits great generalization abilities in various scenarios, enabling real-time perception even in environments exceeding 1000 square meters.
* 11 pages, 6 figures
Via
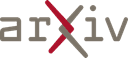
Jun 18, 2025
Abstract:Camouflaged object detection (COD) primarily focuses on learning subtle yet discriminative representations from complex scenes. Existing methods predominantly follow the parametric feedforward architecture based on static visual representation modeling. However, they lack explicit mechanisms for acquiring historical context, limiting their adaptation and effectiveness in handling challenging camouflage scenes. In this paper, we propose a recall-augmented COD architecture, namely RetroMem, which dynamically modulates camouflage pattern perception and inference by integrating relevant historical knowledge into the process. Specifically, RetroMem employs a two-stage training paradigm consisting of a learning stage and a recall stage to construct, update, and utilize memory representations effectively. During the learning stage, we design a dense multi-scale adapter (DMA) to improve the pretrained encoder's capability to capture rich multi-scale visual information with very few trainable parameters, thereby providing foundational inferences. In the recall stage, we propose a dynamic memory mechanism (DMM) and an inference pattern reconstruction (IPR). These components fully leverage the latent relationships between learned knowledge and current sample context to reconstruct the inference of camouflage patterns, thereby significantly improving the model's understanding of camouflage scenes. Extensive experiments on several widely used datasets demonstrate that our RetroMem significantly outperforms existing state-of-the-art methods.
Via
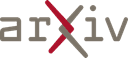
Jun 18, 2025
Abstract:This paper presents a generalization of the trajectory general optimal sub-pattern assignment (GOSPA) metric for evaluating multi-object tracking algorithms that provide trajectory estimates with track-level uncertainties. This metric builds on the recently introduced probabilistic GOSPA metric to account for both the existence and state estimation uncertainties of individual object states. Similar to trajectory GOSPA (TGOSPA), it can be formulated as a multidimensional assignment problem, and its linear programming relaxation--also a valid metric--is computable in polynomial time. Additionally, this metric retains the interpretability of TGOSPA, and we show that its decomposition yields intuitive costs terms associated to expected localization error and existence probability mismatch error for properly detected objects, expected missed and false detection error, and track switch error. The effectiveness of the proposed metric is demonstrated through a simulation study.
* 7 pages, 4 figures
Via
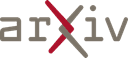
Jun 17, 2025
Abstract:In general, background subtraction-based methods are used to detect moving objects in visual tracking applications. In this paper, we employed a background subtraction-based scheme to detect the temporarily stationary objects. We proposed two schemes for stationary object detection, and we compare those in terms of detection performance and computational complexity. In the first approach, we used a single background, and in the second approach, we used dual backgrounds, generated with different learning rates, in order to detect temporarily stopped objects. Finally, we used normalized cross correlation (NCC) based image comparison to monitor and track the detected stationary object in a video scene. The proposed method is robust with partial occlusion, short-time fully occlusion, and illumination changes, and it can operate in real time.
* Smart Media Journal 1 (2012) 48-55
* 8 pages, 6 figures
Via
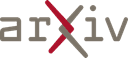