What is Food Recognition? Food recognition is the process of identifying and categorizing different types of food in images or videos.
Papers and Code
Apr 09, 2025
Abstract:Automatic dietary assessment based on food images remains a challenge, requiring precise food detection, segmentation, and classification. Vision-Language Models (VLMs) offer new possibilities by integrating visual and textual reasoning. In this study, we evaluate six state-of-the-art VLMs (ChatGPT, Gemini, Claude, Moondream, DeepSeek, and LLaVA), analyzing their capabilities in food recognition at different levels. For the experimental framework, we introduce the FoodNExTDB, a unique food image database that contains 9,263 expert-labeled images across 10 categories (e.g., "protein source"), 62 subcategories (e.g., "poultry"), and 9 cooking styles (e.g., "grilled"). In total, FoodNExTDB includes 50k nutritional labels generated by seven experts who manually annotated all images in the database. Also, we propose a novel evaluation metric, Expert-Weighted Recall (EWR), that accounts for the inter-annotator variability. Results show that closed-source models outperform open-source ones, achieving over 90% EWR in recognizing food products in images containing a single product. Despite their potential, current VLMs face challenges in fine-grained food recognition, particularly in distinguishing subtle differences in cooking styles and visually similar food items, which limits their reliability for automatic dietary assessment. The FoodNExTDB database is publicly available at https://github.com/AI4Food/FoodNExtDB.
* Accepted at IEEE/CVF Computer Vision and Pattern Recognition
Conference workshops 2025 (CVPRw) 10 pages, 4 figures, 2 tables
Via
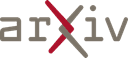
Apr 14, 2025
Abstract:Fine-Grained Image Classification (FGIC) remains a complex task in computer vision, as it requires models to distinguish between categories with subtle localized visual differences. Well-studied CNN-based models, while strong in local feature extraction, often fail to capture the global context required for fine-grained recognition, while more recent ViT-backboned models address FGIC with attention-driven mechanisms but lack the ability to adaptively focus on truly discriminative regions. TransFG and other ViT-based extensions introduced part-aware token selection to enhance attention localization, yet they still struggle with computational efficiency, attention region selection flexibility, and detail-focus narrative in complex environments. This paper introduces GFT (Gradient Focal Transformer), a new ViT-derived framework created for FGIC tasks. GFT integrates the Gradient Attention Learning Alignment (GALA) mechanism to dynamically prioritize class-discriminative features by analyzing attention gradient flow. Coupled with a Progressive Patch Selection (PPS) strategy, the model progressively filters out less informative regions, reducing computational overhead while enhancing sensitivity to fine details. GFT achieves SOTA accuracy on FGVC Aircraft, Food-101, and COCO datasets with 93M parameters, outperforming ViT-based advanced FGIC models in efficiency. By bridging global context and localized detail extraction, GFT sets a new benchmark in fine-grained recognition, offering interpretable solutions for real-world deployment scenarios.
* 11 pages, 3 tables, 5 figures
Via
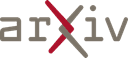
Mar 24, 2025
Abstract:Food recognition models often struggle to distinguish between seen and unseen samples, frequently misclassifying samples from unseen categories by assigning them an in-distribution (ID) label. This misclassification presents significant challenges when deploying these models in real-world applications, particularly within automatic dietary assessment systems, where incorrect labels can lead to cascading errors throughout the system. Ideally, such models should prompt the user when an unknown sample is encountered, allowing for corrective action. Given no prior research exploring food recognition in real-world settings, in this work we conduct an empirical analysis of various post-hoc out-of-distribution (OOD) detection methods for fine-grained food recognition. Our findings indicate that virtual logit matching (ViM) performed the best overall, likely due to its combination of logits and feature-space representations. Additionally, our work reinforces prior notions in the OOD domain, noting that models with higher ID accuracy performed better across the evaluated OOD detection methods. Furthermore, transformer-based architectures consistently outperformed convolution-based models in detecting OOD samples across various methods.
Via
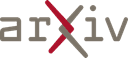
Mar 15, 2025
Abstract:In recent years, Transformer has witnessed significant progress in food recognition. However, most existing approaches still face two critical challenges in lightweight food recognition: (1) the quadratic complexity and redundant feature representation from interactions with irrelevant tokens; (2) static feature recognition and single-scale representation, which overlook the unstructured, non-fixed nature of food images and the need for multi-scale features. To address these, we propose an adaptive and efficient sparse Transformer architecture (Fraesormer) with two core designs: Adaptive Top-k Sparse Partial Attention (ATK-SPA) and Hierarchical Scale-Sensitive Feature Gating Network (HSSFGN). ATK-SPA uses a learnable Gated Dynamic Top-K Operator (GDTKO) to retain critical attention scores, filtering low query-key matches that hinder feature aggregation. It also introduces a partial channel mechanism to reduce redundancy and promote expert information flow, enabling local-global collaborative modeling. HSSFGN employs gating mechanism to achieve multi-scale feature representation, enhancing contextual semantic information. Extensive experiments show that Fraesormer outperforms state-of-the-art methods. code is available at https://zs1314.github.io/Fraesormer.
* 6 pages, 4 figures
Via
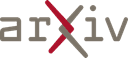
Mar 14, 2025
Abstract:Plankton recognition is an important computer vision problem due to plankton's essential role in ocean food webs and carbon capture, highlighting the need for species-level monitoring. However, this task is challenging due to its fine-grained nature and dataset shifts caused by different imaging instruments and varying species distributions. As new plankton image datasets are collected at an increasing pace, there is a need for general plankton recognition models that require minimal expert effort for data labeling. In this work, we study large-scale self-supervised pretraining for fine-grained plankton recognition. We first employ masked autoencoding and a large volume of diverse plankton image data to pretrain a general-purpose plankton image encoder. Then we utilize fine-tuning to obtain accurate plankton recognition models for new datasets with a very limited number of labeled training images. Our experiments show that self-supervised pretraining with diverse plankton data clearly increases plankton recognition accuracy compared to standard ImageNet pretraining when the amount of training data is limited. Moreover, the accuracy can be further improved when unlabeled target data is available and utilized during the pretraining.
Via
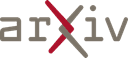
Mar 14, 2025
Abstract:This paper considers open-set recognition (OSR) of plankton images. Plankton include a diverse range of microscopic aquatic organisms that have an important role in marine ecosystems as primary producers and as a base of food webs. Given their sensitivity to environmental changes, fluctuations in plankton populations offer valuable information about oceans' health and climate change motivating their monitoring. Modern automatic plankton imaging devices enable the collection of large-scale plankton image datasets, facilitating species-level analysis. Plankton species recognition can be seen as an image classification task and is typically solved using deep learning-based image recognition models. However, data collection in real aquatic environments results in imaging devices capturing a variety of non-plankton particles and plankton species not present in the training set. This creates a challenging fine-grained OSR problem, characterized by subtle differences between taxonomically close plankton species. We address this challenge by conducting extensive experiments on three OSR approaches using both phyto- and zooplankton images analyzing also on the effect of the rejection thresholds for OSR. The results demonstrate that high OSR accuracy can be obtained promoting the use of these methods in operational plankton research. We have made the data publicly available to the research community.
* ECCV 2024, OOD-CV workshop paper
Via
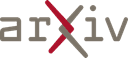
Feb 24, 2025
Abstract:The integration of photovoltaic (PV) systems into greenhouses not only optimizes land use but also enhances sustainable agricultural practices by enabling dual benefits of food production and renewable energy generation. However, accurate prediction of internal environmental conditions is crucial to ensure optimal crop growth while maximizing energy production. This study introduces a novel application of Spatio-Temporal Graph Neural Networks (STGNNs) to greenhouse microclimate modeling, comparing their performance with traditional Recurrent Neural Networks (RNNs). While RNNs excel at temporal pattern recognition, they cannot explicitly model the directional relationships between environmental variables. Our STGNN approach addresses this limitation by representing these relationships as directed graphs, enabling the model to capture both spatial dependencies and their directionality. Using high-frequency data collected at 15-minute intervals from a greenhouse in Volos, Greece, we demonstrate that RNNs achieve exceptional accuracy in winter conditions (R^2 = 0.985) but show limitations during summer cooling system operation. Though STGNNs currently show lower performance (winter R^2 = 0.947), their architecture offers greater potential for integrating additional variables such as PV generation and crop growth indicators.
Via
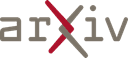
Dec 13, 2024
Abstract:The obesity phenomenon, known as the heavy issue, is a leading cause of preventable chronic diseases worldwide. Traditional calorie estimation tools often rely on specific data formats or complex pipelines, limiting their practicality in real-world scenarios. Recently, vision-language models (VLMs) have excelled in understanding real-world contexts and enabling conversational interactions, making them ideal for downstream tasks such as ingredient analysis. However, applying VLMs to calorie estimation requires domain-specific data and alignment strategies. To this end, we curated CalData, a 330K image-text pair dataset tailored for ingredient recognition and calorie estimation, combining a large-scale recipe dataset with detailed nutritional instructions for robust vision-language training. Built upon this dataset, we present CaLoRAify, a novel VLM framework aligning ingredient recognition and calorie estimation via training with visual-text pairs. During inference, users only need a single monocular food image to estimate calories while retaining the flexibility of agent-based conversational interaction. With Low-rank Adaptation (LoRA) and Retrieve-augmented Generation (RAG) techniques, our system enhances the performance of foundational VLMs in the vertical domain of calorie estimation. Our code and data are fully open-sourced at https://github.com/KennyYao2001/16824-CaLORAify.
* Disclaimer: This work is part of a course project and reflects
ongoing exploration in the field of vision-language models and calorie
estimation. Findings and conclusions are subject to further validation and
refinement
Via
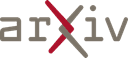
Dec 09, 2024
Abstract:This research builds upon the Latvian Twitter Eater Corpus (LTEC), which is focused on the narrow domain of tweets related to food, drinks, eating and drinking. LTEC has been collected for more than 12 years and reaching almost 3 million tweets with the basic information as well as extended automatically and manually annotated metadata. In this paper we supplement the LTEC with manually annotated subsets of evaluation data for machine translation, named entity recognition, timeline-balanced sentiment analysis, and text-image relation classification. We experiment with each of the data sets using baseline models and highlight future challenges for various modelling approaches.
* Proceedings of the 2024 Joint International Conference on
Computational Linguistics, Language Resources and Evaluation (LREC-COLING
2024)
Via
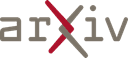
Nov 19, 2024
Abstract:Monitoring agricultural activities is important to ensure food security. Remote sensing plays a significant role for large-scale continuous monitoring of cultivation activities. Time series remote sensing data were used for the generation of the cropping pattern. Classification algorithms are used to classify crop patterns and mapped agriculture land used. Some conventional classification methods including support vector machine (SVM) and decision trees were applied for crop pattern recognition. However, in this paper, we are proposing Deep Neural Network (DNN) based classification to improve the performance of crop pattern recognition and make a comparative analysis with two (2) other machine learning approaches including Naive Bayes and Random Forest.
* Published in ICNTET2018: International Conference on New Trends in
Engineering & Technology Tirupathi Highway, Tiruvallur Dist Chennai, India,
September 7-8, 2018
Via
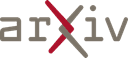