Topic:Face Beautification
What is Face Beautification? Face beautification is the process of enhancing the appearance of a person's face in images or videos.
Papers and Code
Apr 12, 2024
Abstract:With the advent of social media, fun selfie filters have come into tremendous mainstream use affecting the functioning of facial biometric systems as well as image recognition systems. These filters vary from beautification filters and Augmented Reality (AR)-based filters to filters that modify facial landmarks. Hence, there is a need to assess the impact of such filters on the performance of existing face recognition systems. The limitation associated with existing solutions is that these solutions focus more on the beautification filters. However, the current AR-based filters and filters which distort facial key points are in vogue recently and make the faces highly unrecognizable even to the naked eye. Also, the filters considered are mostly obsolete with limited variations. To mitigate these limitations, we aim to perform a holistic impact analysis of the latest filters and propose an user recognition model with the filtered images. We have utilized a benchmark dataset for baseline images, and applied the latest filters over them to generate a beautified/filtered dataset. Next, we have introduced a model FaceFilterNet for beautified user recognition. In this framework, we also utilize our model to comment on various attributes of the person including age, gender, and ethnicity. In addition, we have also presented a filter-wise impact analysis on face recognition, age estimation, gender, and ethnicity prediction. The proposed method affirms the efficacy of our dataset with an accuracy of 87.25% and an optimal accuracy for facial attribute analysis.
Via
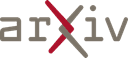
May 02, 2023
Abstract:Recently, due to COVID-19 and the growing demand for remote work, video conferencing apps have become especially widespread. The most valuable features of video chats are real-time background removal and face beautification. While solving these tasks, computer vision researchers face the problem of having relevant data for the training stage. There is no large dataset with high-quality labeled and diverse images of people in front of a laptop or smartphone camera to train a lightweight model without additional approaches. To boost the progress in this area, we provide a new image dataset, EasyPortrait, for portrait segmentation and face parsing tasks. It contains 20,000 primarily indoor photos of 8,377 unique users, and fine-grained segmentation masks separated into 9 classes. Images are collected and labeled from crowdsourcing platforms. Unlike most face parsing datasets, in EasyPortrait, the beard is not considered part of the skin mask, and the inside area of the mouth is separated from the teeth. These features allow using EasyPortrait for skin enhancement and teeth whitening tasks. This paper describes the pipeline for creating a large-scale and clean image segmentation dataset using crowdsourcing platforms without additional synthetic data. Moreover, we trained several models on EasyPortrait and showed experimental results. Proposed dataset and trained models are publicly available.
* portrait segmentation, face parsing, image segmentation dataset
Via
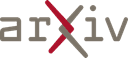
Mar 01, 2023
Abstract:Augmented reality and other photo editing filters are popular methods used to modify images, especially images of faces, posted online. Considering the important role of human facial perception in social communication, how does exposure to an increasing number of modified faces online affect human facial perception? In this paper we present the results of six surveys designed to measure familiarity with different styles of facial filters, perceived strangeness of faces edited with different facial filters, and ability to discern whether images are filtered or not. Our results indicate that faces filtered with photo editing filters that change the image color tones, modify facial structure, or add facial beautification tend to be perceived similarly to unmodified faces; however, faces filtered with augmented reality filters (\textit{i.e.,} filters that overlay digital objects) are perceived differently from unmodified faces. We also found that responses differed based on different survey question phrasings, indicating that the shift in facial perception due to the prevalence of filtered images is noisy to detect. A better understanding of shifts in facial perception caused by facial filters will help us build online spaces more responsibly and could inform the training of more accurate and equitable facial recognition models, especially those trained with human psychophysical annotations.
Via
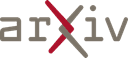
Dec 19, 2022
Abstract:Recent years have witnessed significant growth of face alignment. Though dense facial landmark is highly demanded in various scenarios, e.g., cosmetic medicine and facial beautification, most works only consider sparse face alignment. To address this problem, we present a framework that can enrich landmark density by existing sparse landmark datasets, e.g., 300W with 68 points and WFLW with 98 points. Firstly, we observe that the local patches along each semantic contour are highly similar in appearance. Then, we propose a weakly-supervised idea of learning the refinement ability on original sparse landmarks and adapting this ability to enriched dense landmarks. Meanwhile, several operators are devised and organized together to implement the idea. Finally, the trained model is applied as a plug-and-play module to the existing face alignment networks. To evaluate our method, we manually label the dense landmarks on 300W testset. Our method yields state-of-the-art accuracy not only in newly-constructed dense 300W testset but also in the original sparse 300W and WFLW testsets without additional cost.
* AAAI 2023
Via
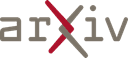
Mar 11, 2022
Abstract:Selfie images enjoy huge popularity in social media. The same platforms centered around sharing this type of images offer filters to beautify them or incorporate augmented reality effects. Studies suggests that filtered images attract more views and engagement. Selfie images are also in increasing use in security applications due to mobiles becoming data hubs for many transactions. Also, video conference applications, boomed during the pandemic, include such filters. Such filters may destroy biometric features that would allow person recognition or even detection of the face itself, even if such commodity applications are not necessarily used to compromise facial systems. This could also affect subsequent investigations like crimes in social media, where automatic analysis is usually necessary given the amount of information posted in social sites or stored in devices or cloud repositories. To help in counteracting such issues, we contribute with a database of facial images that includes several manipulations. It includes image enhancement filters (which mostly modify contrast and lightning) and augmented reality filters that incorporate items like animal noses or glasses. Additionally, images with sunglasses are processed with a reconstruction network trained to learn to reverse such modifications. This is because obfuscating the eye region has been observed in the literature to have the highest impact on the accuracy of face detection or recognition. We start from the popular Labeled Faces in the Wild (LFW) database, to which we apply different modifications, generating 8 datasets. Each dataset contains 4,324 images of size 64 x 64, with a total of 34,592 images. The use of a public and widely employed face dataset allows for replication and comparison. The created database is available at https://github.com/HalmstadUniversityBiometrics/LFW-Beautified
* Under consideration at Elsevier Data in Brief
Via
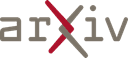
Oct 20, 2021
Abstract:Beautification and augmented reality filters are very popular in applications that use selfie images captured with smartphones or personal devices. However, they can distort or modify biometric features, severely affecting the capability of recognizing individuals' identity or even detecting the face. Accordingly, we address the effect of such filters on the accuracy of automated face detection and recognition. The social media image filters studied either modify the image contrast or illumination or occlude parts of the face with for example artificial glasses or animal noses. We observe that the effect of some of these filters is harmful both to face detection and identity recognition, specially if they obfuscate the eye or (to a lesser extent) the nose. To counteract such effect, we develop a method to reconstruct the applied manipulation with a modified version of the U-NET segmentation network. This is observed to contribute to a better face detection and recognition accuracy. From a recognition perspective, we employ distance measures and trained machine learning algorithms applied to features extracted using a ResNet-34 network trained to recognize faces. We also evaluate if incorporating filtered images to the training set of machine learning approaches are beneficial for identity recognition. Our results show good recognition when filters do not occlude important landmarks, specially the eyes (identification accuracy >99%, EER<2%). The combined effect of the proposed approaches also allow to mitigate the effect produced by filters that occlude parts of the face, achieving an identification accuracy of >92% with the majority of perturbations evaluated, and an EER <8%. Although there is room for improvement, when neither U-NET reconstruction nor training with filtered images is applied, the accuracy with filters that severely occlude the eye is <72% (identification) and >12% (EER)
Via
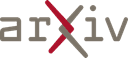
Dec 11, 2019
Abstract:Facial appearance plays an important role in our social lives. Subjective perception of women's beauty depends on various face-related (e.g., skin, shape, hair) and environmental (e.g., makeup, lighting, angle) factors. Similar to cosmetic surgery in the physical world, virtual face beautification is an emerging field with many open issues to be addressed. Inspired by the latest advances in style-based synthesis and face beauty prediction, we propose a novel framework of face beautification. For a given reference face with a high beauty score, our GAN-based architecture is capable of translating an inquiry face into a sequence of beautified face images with referenced beauty style and targeted beauty score values. To achieve this objective, we propose to integrate both style-based beauty representation (extracted from the reference face) and beauty score prediction (trained on SCUT-FBP database) into the process of beautification. Unlike makeup transfer, our approach targets at many-to-many (instead of one-to-one) translation where multiple outputs can be defined by either different references or varying beauty scores. Extensive experimental results are reported to demonstrate the effectiveness and flexibility of the proposed face beautification framework.
Via
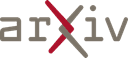
Dec 17, 2019
Abstract:This paper tackles unpaired image enhancement, a task of learning a mapping function which transforms input images into enhanced images in the absence of input-output image pairs. Our method is based on generative adversarial networks (GANs), but instead of simply generating images with a neural network, we enhance images utilizing image editing software such as Adobe Photoshop for the following three benefits: enhanced images have no artifacts, the same enhancement can be applied to larger images, and the enhancement is interpretable. To incorporate image editing software into a GAN, we propose a reinforcement learning framework where the generator works as the agent that selects the software's parameters and is rewarded when it fools the discriminator. Our framework can use high-quality non-differentiable filters present in image editing software, which enables image enhancement with high performance. We apply the proposed method to two unpaired image enhancement tasks: photo enhancement and face beautification. Our experimental results demonstrate that the proposed method achieves better performance, compared to the performances of the state-of-the-art methods based on unpaired learning.
* Accepted to AAAI 2020
Via
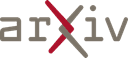
Feb 25, 2019
Abstract:Beauty is in the eye of the beholder. This maxim, emphasizing the subjectivity of the perception of beauty, has enjoyed a wide consensus since ancient times. In the digitalera, data-driven methods have been shown to be able to predict human-assigned beauty scores for facial images. In this work, we augment this ability and train a generative model that generates faces conditioned on a requested beauty score. In addition, we show how this trained generator can be used to beautify an input face image. By doing so, we achieve an unsupervised beautification model, in the sense that it relies on no ground truth target images.
Via
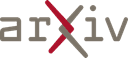