Feb 10, 2025
Abstract:Machine learning is a vital part of many real-world systems, but several concerns remain about the lack of interpretability, explainability and robustness of black-box AI systems. Concept-based models (CBM) address some of these challenges by learning interpretable concepts from high-dimensional data, e.g. images, which are used to predict labels. An important issue in CBMs is concept leakage, i.e., spurious information in the learned concepts, which effectively leads to learning "wrong" concepts. Current mitigating strategies are heuristic, have strong assumptions, e.g., they assume that the concepts are statistically independent of each other, or require substantial human interaction in terms of both interventions and labels provided by annotators. In this paper, we describe a framework that provides theoretical guarantees on the correctness of the learned concepts and on the number of required labels, without requiring any interventions. Our framework leverages causal representation learning (CRL) to learn high-level causal variables from low-level data, and learns to align these variables with interpretable concepts. We propose a linear and a non-parametric estimator for this mapping, providing a finite-sample high probability result in the linear case and an asymptotic consistency result for the non-parametric estimator. We implement our framework with state-of-the-art CRL methods, and show its efficacy in learning the correct concepts in synthetic and image benchmarks.
* 47 pages, 16 figures, 9 Tables, Preprint
Via
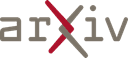