Dec 21, 2024
Abstract:This paper proposes a novel approach to develop an open-domain and long-form Over-The-Top (OTT) Question-Answering (QA) dataset, DragonVerseQA, specifically oriented to the fantasy universe of "House of the Dragon" and "Game Of Thrones" TV series. Most existing QA datasets focus on short, fact-based answers sourced almost solely from Wikipedia articles, devoid of depth and contextual richness for sophisticated narrative understanding. We curate a dataset that combines full episode summaries sourced from HBO and fandom wiki websites, user reviews from sources like IMDb and Rotten Tomatoes, and high-quality, open-domain, legally admissible sources, and structured data from repositories like WikiData into one dataset. The dataset provides a multi-dimensional context, reflecting complex character dynamics and plot developments from these varied sources. That means, on equal footing, only after heavy data preprocessing and filtering methods will meaningful, non-spam unbiased reviews be available in this enriched dataset. The comprehensive insights are given through the long-form answers generated from this enriched context. This is what makes this valuable dataset for improving conversational AI, narrative analysis, sentiment analysis, summarization techniques, and relation extraction. A comparative analysis with state-of-the-art QA datasets such as SQuAD 2.0, TriviaQA, and Natural Questions brings to light the unique advantages of our dataset in terms of contextual complexity and answer length. Detailed reviews add layers to audience sentiment and narrative interpretation, raising the bar for domain-specific QA with a new quality benchmark. Our work also allows a deeper understanding of entertainment-industry content and opens the door to more knowledgeable and creative AI-driven interactions within digital media environments.
Via
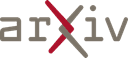