Oct 31, 2024
Abstract:This study presents a comprehensive benchmark analysis of various YOLO (You Only Look Once) algorithms, from YOLOv3 to the newest addition. It represents the first research to comprehensively evaluate the performance of YOLO11, the latest addition to the YOLO family. It evaluates their performance on three diverse datasets: Traffic Signs (with varying object sizes), African Wildlife (with diverse aspect ratios and at least one instance of the object per image), and Ships and Vessels (with small-sized objects of a single class), ensuring a comprehensive assessment across datasets with distinct challenges. To ensure a robust evaluation, we employ a comprehensive set of metrics, including Precision, Recall, Mean Average Precision (mAP), Processing Time, GFLOPs count, and Model Size. Our analysis highlights the distinctive strengths and limitations of each YOLO version. For example: YOLOv9 demonstrates substantial accuracy but struggles with detecting small objects and efficiency whereas YOLOv10 exhibits relatively lower accuracy due to architectural choices that affect its performance in overlapping object detection but excels in speed and efficiency. Additionally, the YOLO11 family consistently shows superior performance in terms of accuracy, speed, computational efficiency, and model size. YOLO11m achieved a remarkable balance of accuracy and efficiency, scoring mAP50-95 scores of 0.795, 0.81, and 0.325 on the Traffic Signs, African Wildlife, and Ships datasets, respectively, while maintaining an average inference time of 2.4ms, a model size of 38.8Mb, and around 67.6 GFLOPs on average. These results provide critical insights for both industry and academia, facilitating the selection of the most suitable YOLO algorithm for diverse applications and guiding future enhancements.
* 20 pages
Via
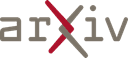