Topic:Text Extraction From Documents
What is Text Extraction From Documents? Text extraction from documents is the process of extracting text data from scanned documents or images.
Papers and Code
Mar 20, 2025
Abstract:The automated analysis of chemical literature holds promise to accelerate discovery in fields such as material science and drug development. In particular, search capabilities for chemical structures and Markush structures (chemical structure templates) within patent documents are valuable, e.g., for prior-art search. Advancements have been made in the automatic extraction of chemical structures from text and images, yet the Markush structures remain largely unexplored due to their complex multi-modal nature. In this work, we present MarkushGrapher, a multi-modal approach for recognizing Markush structures in documents. Our method jointly encodes text, image, and layout information through a Vision-Text-Layout encoder and an Optical Chemical Structure Recognition vision encoder. These representations are merged and used to auto-regressively generate a sequential graph representation of the Markush structure along with a table defining its variable groups. To overcome the lack of real-world training data, we propose a synthetic data generation pipeline that produces a wide range of realistic Markush structures. Additionally, we present M2S, the first annotated benchmark of real-world Markush structures, to advance research on this challenging task. Extensive experiments demonstrate that our approach outperforms state-of-the-art chemistry-specific and general-purpose vision-language models in most evaluation settings. Code, models, and datasets will be available.
Via
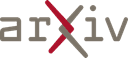
Mar 21, 2025
Abstract:Visual Information Extraction (VIE), aiming at extracting structured information from visually rich document images, plays a pivotal role in document processing. Considering various layouts, semantic scopes, and languages, VIE encompasses an extensive range of types, potentially numbering in the thousands. However, many of these types suffer from a lack of training data, which poses significant challenges. In this paper, we propose a novel generative model, named Generative Compositor, to address the challenge of few-shot VIE. The Generative Compositor is a hybrid pointer-generator network that emulates the operations of a compositor by retrieving words from the source text and assembling them based on the provided prompts. Furthermore, three pre-training strategies are employed to enhance the model's perception of spatial context information. Besides, a prompt-aware resampler is specially designed to enable efficient matching by leveraging the entity-semantic prior contained in prompts. The introduction of the prompt-based retrieval mechanism and the pre-training strategies enable the model to acquire more effective spatial and semantic clues with limited training samples. Experiments demonstrate that the proposed method achieves highly competitive results in the full-sample training, while notably outperforms the baseline in the 1-shot, 5-shot, and 10-shot settings.
Via
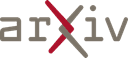
Mar 19, 2025
Abstract:Background: Patient recruitment in clinical trials is hindered by complex eligibility criteria and labor-intensive chart reviews. Prior research using text-only models have struggled to address this problem in a reliable and scalable way due to (1) limited reasoning capabilities, (2) information loss from converting visual records to text, and (3) lack of a generic EHR integration to extract patient data. Methods: We introduce a broadly applicable, integration-free, LLM-powered pipeline that automates patient-trial matching using unprocessed documents extracted from EHRs. Our approach leverages (1) the new reasoning-LLM paradigm, enabling the assessment of even the most complex criteria, (2) visual capabilities of latest LLMs to interpret medical records without lossy image-to-text conversions, and (3) multimodal embeddings for efficient medical record search. The pipeline was validated on the n2c2 2018 cohort selection dataset (288 diabetic patients) and a real-world dataset composed of 485 patients from 30 different sites matched against 36 diverse trials. Results: On the n2c2 dataset, our method achieved a new state-of-the-art criterion-level accuracy of 93\%. In real-world trials, the pipeline yielded an accuracy of 87\%, undermined by the difficulty to replicate human decision-making when medical records lack sufficient information. Nevertheless, users were able to review overall eligibility in under 9 minutes per patient on average, representing an 80\% improvement over traditional manual chart reviews. Conclusion: This pipeline demonstrates robust performance in clinical trial patient matching without requiring custom integration with site systems or trial-specific tailoring, thereby enabling scalable deployment across sites seeking to leverage AI for patient matching.
Via
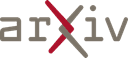
Mar 20, 2025
Abstract:Remote work and online courses have become important methods of knowledge dissemination, leading to a large number of document-based instructional videos. Unlike traditional video datasets, these videos mainly feature rich-text images and audio that are densely packed with information closely tied to the visual content, requiring advanced multimodal understanding capabilities. However, this domain remains underexplored due to dataset availability and its inherent complexity. In this paper, we introduce the DocVideoQA task and dataset for the first time, comprising 1454 videos across 23 categories with a total duration of about 828 hours. The dataset is annotated with 154k question-answer pairs generated manually and via GPT, assessing models' comprehension, temporal awareness, and modality integration capabilities. Initially, we establish a baseline using open-source MLLMs. Recognizing the challenges in modality comprehension for document-centric videos, we present DV-LLaMA, a robust video MLLM baseline. Our method enhances unimodal feature extraction with diverse instruction-tuning data and employs contrastive learning to strengthen modality integration. Through fine-tuning, the LLM is equipped with audio-visual capabilities, leading to significant improvements in document-centric video understanding. Extensive testing on the DocVideoQA dataset shows that DV-LLaMA significantly outperforms existing models. We'll release the code and dataset to facilitate future research.
Via
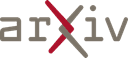
Mar 19, 2025
Abstract:Traditional information retrieval is primarily concerned with finding relevant information from large datasets without imposing a structure within the retrieved pieces of data. However, structuring information in the form of narratives--ordered sets of documents that form coherent storylines--allows us to identify, interpret, and share insights about the connections and relationships between the ideas presented in the data. Despite their significance, current approaches for algorithmically extracting storylines from data are scarce, with existing methods primarily relying on intricate word-based heuristics and auxiliary document structures. Moreover, many of these methods are difficult to scale to large datasets and general contexts, as they are designed to extract storylines for narrow tasks. In this paper, we propose Narrative Trails, an efficient, general-purpose method for extracting coherent storylines in large text corpora. Specifically, our method uses the semantic-level information embedded in the latent space of deep learning models to build a sparse coherence graph and extract narratives that maximize the minimum coherence of the storylines. By quantitatively evaluating our proposed methods on two distinct narrative extraction tasks, we show the generalizability and scalability of Narrative Trails in multiple contexts while also simplifying the extraction pipeline.
* Eighth Text2Story Workshop at the 47th European Conference on
Information Retrieval (ECIR 2025). The code for our algorithm, evaluations,
and examples are available at
https://github.com/faustogerman/narrative-trails
Via
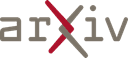
Mar 18, 2025
Abstract:Cardiopulmonary exercise testing (CPET) provides a comprehensive assessment of functional capacity by measuring key physiological variables including oxygen consumption ($VO_2$), carbon dioxide production ($VCO_2$), and pulmonary ventilation ($VE$) during exercise. Previous research has established that parameters such as peak $VO_2$ and $VE/VCO_2$ ratio serve as robust predictors of mortality risk in chronic heart failure patients. In this study, we leverage CPET variables as surrogate mortality endpoints for patients with Congenital Heart Disease (CHD). To our knowledge, this represents the first successful implementation of an advanced machine learning approach that predicts CPET outcomes by integrating electrocardiograms (ECGs) with information derived from clinical letters. Our methodology began with extracting unstructured patient information-including intervention history, diagnoses, and medication regimens-from clinical letters using natural language processing techniques, organizing this data into a structured database. We then digitized ECGs to obtain quantifiable waveforms and established comprehensive data linkages. The core innovation of our approach lies in exploiting the Riemannian geometric properties of covariance matrices derived from both 12-lead ECGs and clinical text data to develop robust regression and classification models. Through extensive ablation studies, we demonstrated that the integration of ECG signals with clinical documentation, enhanced by covariance augmentation techniques in Riemannian space, consistently produced superior predictive performance compared to conventional approaches.
* preprint for Scientific Reports
Via
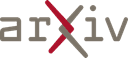
Mar 15, 2025
Abstract:Large Language Models (LLMs) have revolutionized natural language processing through their state of art reasoning capabilities. This paper explores the convergence of LLM reasoning techniques and feature generation for machine learning tasks. We examine four key reasoning approaches: Chain of Thought, Tree of Thoughts, Retrieval-Augmented Generation, and Thought Space Exploration. Our analysis reveals how these approaches can be used to identify effective feature generation rules without having to manually specify search spaces. The paper categorizes LLM-based feature generation methods across various domains including finance, healthcare, and text analytics. LLMs can extract key information from clinical notes and radiology reports in healthcare, by enabling more efficient data utilization. In finance, LLMs facilitate text generation, summarization, and entity extraction from complex documents. We analyze evaluation methodologies for assessing feature quality and downstream performance, with particular attention to OCTree's decision tree reasoning approach that provides language-based feedback for iterative improvements. Current challenges include hallucination, computational efficiency, and domain adaptation. As of March 2025, emerging approaches include inference-time compute scaling, reinforcement learning, and supervised fine-tuning with model distillation. Future directions point toward multimodal feature generation, self-improving systems, and neuro-symbolic approaches. This paper provides a detailed overview of an emerging field that promises to automate and enhance feature engineering through language model reasoning.
Via
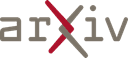
Mar 13, 2025
Abstract:The increasing volume of textual data poses challenges in reading and comprehending large documents, particularly for scholars who need to extract useful information from research articles. Automatic text summarization has emerged as a powerful tool to condense lengthy documents into concise and informative summaries. Depending on the approach used, text summarization can be categorized as either extractive or abstractive. While extractive methods are commonly used due to their simplicity, they often miss important information. On the other hand, Abstractive Summarization can generate more coherent and informative summaries by understanding the underlying meaning of the text. Abstractive techniques have gained attention in various languages, and recent advancements have been achieved through pre-training models such as BERT, BART, and T5. However, the challenge of summarizing long documents remains, and alternative models like Longformer have been introduced to address this limitation. In this context, this paper focuses on abstractive summarization in the Persian language. The authors introduce a new dataset of 300,000 full-text Persian papers obtained from the Ensani website and apply the ARMAN model, based on the Longformer architecture, to generate summaries. The experimental results demonstrate promising performance in Persian text summarization. The paper provides a comprehensive overview of related work, discusses the methodology, presents the experimental results, and concludes with future research directions.
* 11 pages, 3 tables
Via
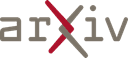
Mar 13, 2025
Abstract:Automatic patent summarization approaches that help in the patent analysis and comprehension procedure are in high demand due to the colossal growth of innovations. The development of natural language processing (NLP), text mining, and deep learning has notably amplified the efficacy of text summarization models for abundant types of documents. Summarizing patent text remains a pertinent challenge due to the labyrinthine writing style of these documents, which includes technical and legal intricacies. Additionally, these patent document contents are considerably lengthier than archetypal documents, which intricates the process of extracting pertinent information for summarization. Embodying extractive and abstractive text summarization methodologies into a hybrid framework, this study proposes a system for efficiently creating abstractive summaries of patent records. The procedure involves leveraging the LexRank graph-based algorithm to retrieve the important sentences from input parent texts, then utilizing a Bidirectional Auto-Regressive Transformer (BART) model that has been fine-tuned using Low-Ranking Adaptation (LoRA) for producing text summaries. This is accompanied by methodical testing and evaluation strategies. Furthermore, the author employed certain meta-learning techniques to achieve Domain Generalization (DG) of the abstractive component across multiple patent fields.
* Accepted Paper in the 8th International Research Conference on Smart
Computing and Systems Engineering, University of Kelaniya, Sri Lanka.
(Pending Publication)
Via
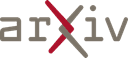
Mar 11, 2025
Abstract:We propose Knowledge-Aware Preprocessing (KAP), a two-stage preprocessing framework tailored for Traditional Chinese non-narrative documents, designed to enhance retrieval accuracy in Hybrid Retrieval systems. Hybrid Retrieval, which integrates Sparse Retrieval (e.g., BM25) and Dense Retrieval (e.g., vector embeddings), has become a widely adopted approach for improving search effectiveness. However, its performance heavily depends on the quality of input text, which is often degraded when dealing with non-narrative documents such as PDFs containing financial statements, contractual clauses, and tables. KAP addresses these challenges by integrating Multimodal Large Language Models (MLLMs) with LLM-driven post-OCR processing, refining extracted text to reduce OCR noise, restore table structures, and optimize text format. By ensuring better compatibility with Hybrid Retrieval, KAP improves the accuracy of both Sparse and Dense Retrieval methods without modifying the retrieval architecture itself.
Via
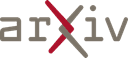