Feb 07, 2025
Abstract:Estimating causal effects from spatiotemporal data is a key challenge in fields such as public health, social policy, and environmental science, where controlled experiments are often infeasible. However, existing causal inference methods relying on observational data face significant limitations: they depend on strong structural assumptions to address spatiotemporal challenges $\unicode{x2013}$ such as interference, spatial confounding, and temporal carryover effects $\unicode{x2013}$ or fail to account for $\textit{time-varying confounders}$. These confounders, influenced by past treatments and outcomes, can themselves shape future treatments and outcomes, creating feedback loops that complicate traditional adjustment strategies. To address these challenges, we introduce the $\textbf{GST-UNet}$ ($\textbf{G}$-computation $\textbf{S}$patio-$\textbf{T}$emporal $\textbf{UNet}$), a novel end-to-end neural network framework designed to estimate treatment effects in complex spatial and temporal settings. The GST-UNet leverages regression-based iterative G-computation to explicitly adjust for time-varying confounders, providing valid estimates of potential outcomes and treatment effects. To the best of our knowledge, the GST-UNet is the first neural model to account for complex, non-linear dynamics and time-varying confounders in spatiotemporal interventions. We demonstrate the effectiveness of the GST-UNet through extensive simulation studies and showcase its practical utility with a real-world analysis of the impact of wildfire smoke on respiratory hospitalizations during the 2018 California Camp Fire. Our results highlight the potential of GST-UNet to advance spatiotemporal causal inference across a wide range of policy-driven and scientific applications.
* 17 pages, 6 figures, 2 tables
Via
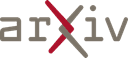