Topic:Joint Entity And Relation Extraction
What is Joint Entity And Relation Extraction? Joint entity-and-relation extraction is the task of extracting entity mentions and semantic relations between entities from unstructured text with a single model.
Papers and Code
Feb 13, 2025
Abstract:Joint entity-relation extraction is a critical task in transforming unstructured or semi-structured text into triplets, facilitating the construction of large-scale knowledge graphs, and supporting various downstream applications. Despite its importance, research on Chinese text, particularly with complex semantics in specialized domains like medicine, remains limited. To address this gap, we introduce the CH-DDI, a Chinese drug-drug interactions dataset designed to capture the intricacies of medical text. Leveraging the strengths of attention mechanisms in capturing long-range dependencies, we propose the SEA module, which enhances the extraction of complex contextual semantic information, thereby improving entity recognition and relation extraction. Additionally, to address the inefficiencies of existing methods in facilitating information exchange between entity recognition and relation extraction, we present an interactive fusion representation module. This module employs Cross Attention for bidirectional information exchange between the tasks and further refines feature extraction through BiLSTM. Experimental results on both our CH-DDI dataset and public CoNLL04 dataset demonstrate that our model exhibits strong generalization capabilities. On the CH-DDI dataset, our model achieves an F1-score of 96.73% for entity recognition and 78.43% for relation extraction. On the CoNLL04 dataset, it attains an entity recognition precision of 89.54% and a relation extraction accuracy of 71.64%.
Via
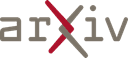
Jan 23, 2025
Abstract:Biological relation networks contain rich information for understanding the biological mechanisms behind the relationship of entities such as genes, proteins, diseases, and chemicals. The vast growth of biomedical literature poses significant challenges updating the network knowledge. The recent Biomedical Relation Extraction Dataset (BioRED) provides valuable manual annotations, facilitating the develop-ment of machine-learning and pre-trained language model approaches for automatically identifying novel document-level (inter-sentence context) relationships. Nonetheless, its annotations lack directionality (subject/object) for the entity roles, essential for studying complex biological networks. Herein we annotate the entity roles of the relationships in the BioRED corpus and subsequently propose a novel multi-task language model with soft-prompt learning to jointly identify the relationship, novel findings, and entity roles. Our results in-clude an enriched BioRED corpus with 10,864 directionality annotations. Moreover, our proposed method outperforms existing large language models such as the state-of-the-art GPT-4 and Llama-3 on two benchmarking tasks. Our source code and dataset are available at https://github.com/ncbi-nlp/BioREDirect.
Via
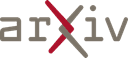
Jan 10, 2025
Abstract:Scene Graph Generation(SGG) is a scene understanding task that aims at identifying object entities and reasoning their relationships within a given image. In contrast to prevailing two-stage methods based on a large object detector (e.g., Faster R-CNN), one-stage methods integrate a fixed-size set of learnable queries to jointly reason relational triplets <subject, predicate, object>. This paradigm demonstrates robust performance with significantly reduced parameters and computational overhead. However, the challenge in one-stage methods stems from the issue of weak entanglement, wherein entities involved in relationships require both coupled features shared within triplets and decoupled visual features. Previous methods either adopt a single decoder for coupled triplet feature modeling or multiple decoders for separate visual feature extraction but fail to consider both. In this paper, we introduce UniQ, a Unified decoder with task-specific Queries architecture, where task-specific queries generate decoupled visual features for subjects, objects, and predicates respectively, and unified decoder enables coupled feature modeling within relational triplets. Experimental results on the Visual Genome dataset demonstrate that UniQ has superior performance to both one-stage and two-stage methods.
* 10 pages, 5 figures
Via
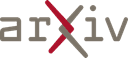
Oct 18, 2024
Abstract:Joint Multimodal Entity-Relation Extraction (JMERE) is a challenging task that aims to extract entities and their relations from text-image pairs in social media posts. Existing methods for JMERE require large amounts of labeled data. However, gathering and annotating fine-grained multimodal data for JMERE poses significant challenges. Initially, we construct diverse and comprehensive multimodal few-shot datasets fitted to the original data distribution. To address the insufficient information in the few-shot setting, we introduce the \textbf{K}nowledge-\textbf{E}nhanced \textbf{C}ross-modal \textbf{P}rompt \textbf{M}odel (KECPM) for JMERE. This method can effectively address the problem of insufficient information in the few-shot setting by guiding a large language model to generate supplementary background knowledge. Our proposed method comprises two stages: (1) a knowledge ingestion stage that dynamically formulates prompts based on semantic similarity guide ChatGPT generating relevant knowledge and employs self-reflection to refine the knowledge; (2) a knowledge-enhanced language model stage that merges the auxiliary knowledge with the original input and utilizes a transformer-based model to align with JMERE's required output format. We extensively evaluate our approach on a few-shot dataset derived from the JMERE dataset, demonstrating its superiority over strong baselines in terms of both micro and macro F$_1$ scores. Additionally, we present qualitative analyses and case studies to elucidate the effectiveness of our model.
* accepted by ACM MM 2024
Via
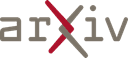
Dec 14, 2024
Abstract:Chinese Named Entity Recognition (NER) is an important task in information extraction, which has a significant impact on downstream applications. Due to the lack of natural separators in Chinese, previous NER methods mostly relied on external dictionaries to enrich the semantic and boundary information of Chinese words. However, such methods may introduce noise that affects the accuracy of named entity recognition. To this end, we propose a character relation enhanced Chinese NER model (CRENER). This model defines four types of tags that reflect the relationships between characters, and proposes a fine-grained modeling of the relationships between characters based on three types of relationships: adjacency relations between characters, relations between characters and tags, and relations between tags, to more accurately identify entity boundaries and improve Chinese NER accuracy. Specifically, we transform the Chinese NER task into a character-character relationship classification task, ensuring the accuracy of entity boundary recognition through joint modeling of relation tags. To enhance the model's ability to understand contextual information, WRENER further constructed an adapted transformer encoder that combines unscaled direction-aware and distance-aware masked self-attention mechanisms. Moreover, a relationship representation enhancement module was constructed to model predefined relationship tags, effectively mining the relationship representations between characters and tags. Experiments conducted on four well-known Chinese NER benchmark datasets have shown that the proposed model outperforms state-of-the-art baselines. The ablation experiment also demonstrated the effectiveness of the proposed model.
Via
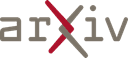
Apr 18, 2024
Abstract:Joint entity and relation extraction plays a pivotal role in various applications, notably in the construction of knowledge graphs. Despite recent progress, existing approaches often fall short in two key aspects: richness of representation and coherence in output structure. These models often rely on handcrafted heuristics for computing entity and relation representations, potentially leading to loss of crucial information. Furthermore, they disregard task and/or dataset-specific constraints, resulting in output structures that lack coherence. In our work, we introduce EnriCo, which mitigates these shortcomings. Firstly, to foster rich and expressive representation, our model leverage attention mechanisms that allow both entities and relations to dynamically determine the pertinent information required for accurate extraction. Secondly, we introduce a series of decoding algorithms designed to infer the highest scoring solutions while adhering to task and dataset-specific constraints, thus promoting structured and coherent outputs. Our model demonstrates competitive performance compared to baselines when evaluated on Joint IE datasets.
* Work in progress
Via
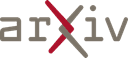
May 14, 2024
Abstract:Named Entity Recognition and Relation Extraction are two crucial and challenging subtasks in the field of Information Extraction. Despite the successes achieved by the traditional approaches, fundamental research questions remain open. First, most recent studies use parameter sharing for a single subtask or shared features for both two subtasks, ignoring their semantic differences. Second, information interaction mainly focuses on the two subtasks, leaving the fine-grained informtion interaction among the subtask-specific features of encoding subjects, relations, and objects unexplored. Motivated by the aforementioned limitations, we propose a novel model to jointly extract entities and relations. The main novelties are as follows: (1) We propose to decouple the feature encoding process into three parts, namely encoding subjects, encoding objects, and encoding relations. Thanks to this, we are able to use fine-grained subtask-specific features. (2) We propose novel inter-aggregation and intra-aggregation strategies to enhance the information interaction and construct individual fine-grained subtask-specific features, respectively. The experimental results demonstrate that our model outperforms several previous state-of-the-art models. Extensive additional experiments further confirm the effectiveness of our model.
Via
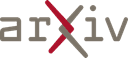
Aug 03, 2024
Abstract:Knowledge graphs (KGs) store enormous facts as relationships between entities. Due to the long-tailed distribution of relations and the incompleteness of KGs, there is growing interest in few-shot knowledge graph completion (FKGC). Existing FKGC methods often assume the existence of all entities in KGs, which may not be practical since new relations and entities can emerge over time. Therefore, we focus on a more challenging task called inductive few-shot knowledge graph completion (I-FKGC), where both relations and entities during the test phase are unknown before. Inspired by the idea of inductive reasoning, we cast I-FKGC as an inductive reasoning problem. Specifically, we propose a novel Graph Stochastic Neural Process approach (GS-NP), which consists of two major modules. In the first module, to obtain a generalized hypothesis (e.g., shared subgraph), we present a neural process-based hypothesis extractor that models the joint distribution of hypothesis, from which we can sample a hypothesis for predictions. In the second module, based on the hypothesis, we propose a graph stochastic attention-based predictor to test if the triple in the query set aligns with the extracted hypothesis. Meanwhile, the predictor can generate an explanatory subgraph identified by the hypothesis. Finally, the training of these two modules is seamlessly combined into a unified objective function, of which the effectiveness is verified by theoretical analyses as well as empirical studies. Extensive experiments on three public datasets demonstrate that our method outperforms existing methods and derives new state-of-the-art performance.
Via
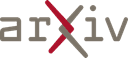
Apr 18, 2024
Abstract:Information extraction (IE) is an important task in Natural Language Processing (NLP), involving the extraction of named entities and their relationships from unstructured text. In this paper, we propose a novel approach to this task by formulating it as graph structure learning (GSL). By formulating IE as GSL, we enhance the model's ability to dynamically refine and optimize the graph structure during the extraction process. This formulation allows for better interaction and structure-informed decisions for entity and relation prediction, in contrast to previous models that have separate or untied predictions for these tasks. When compared against state-of-the-art baselines on joint entity and relation extraction benchmarks, our model, GraphER, achieves competitive results.
* Work in progress
Via
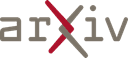
Jan 15, 2024
Abstract:In this paper, we propose a novel method for joint entity and relation extraction from unstructured text by framing it as a conditional sequence generation problem. In contrast to conventional generative information extraction models that are left-to-right token-level generators, our approach is \textit{span-based}. It generates a linearized graph where nodes represent text spans and edges represent relation triplets. Our method employs a transformer encoder-decoder architecture with pointing mechanism on a dynamic vocabulary of spans and relation types. Our model can capture the structural characteristics and boundaries of entities and relations through span representations while simultaneously grounding the generated output in the original text thanks to the pointing mechanism. Evaluation on benchmark datasets validates the effectiveness of our approach, demonstrating competitive results. Code is available at https://github.com/urchade/ATG.
* AAAI 2024 (camera ready version)
Via
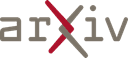