What is Tumor Segmentation? Tumor segmentation is the task of identifying the spatial location of a tumor. It is a pixel-level prediction where each pixel is classified as a tumor or background. The most popular benchmark for this task is the BraTS dataset. The models are typically evaluated with the Dice Score metric.
Papers and Code
Apr 23, 2025
Abstract:In this study, we built an end-to-end tumor-infiltrating lymphocytes (TILs) assessment pipeline within QuPath, demonstrating the potential of easily accessible tools to perform complex tasks in a fully automatic fashion. First, we trained a pixel classifier to segment tumor, tumor-associated stroma, and other tissue compartments in breast cancer H&E-stained whole-slide images (WSI) to isolate tumor-associated stroma for subsequent analysis. Next, we applied a pre-trained StarDist deep learning model in QuPath for cell detection and used the extracted cell features to train a binary classifier distinguishing TILs from other cells. To evaluate our TILs assessment pipeline, we calculated the TIL density in each WSI and categorized them as low, medium, or high TIL levels. Our pipeline was evaluated against pathologist-assigned TIL scores, achieving a Cohen's kappa of 0.71 on the external test set, corroborating previous research findings. These results confirm that existing software can offer a practical solution for the assessment of TILs in H&E-stained WSIs of breast cancer.
* 16 Pages, 9 Figures, 3 tables
Via
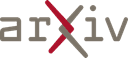
Apr 22, 2025
Abstract:This study performs a comprehensive evaluation of quantitative measurements as extracted from automated deep-learning-based segmentation methods, beyond traditional Dice Similarity Coefficient assessments, focusing on six quantitative metrics, namely SUVmax, SUVmean, total lesion activity (TLA), tumor volume (TMTV), lesion count, and lesion spread. We analyzed 380 prostate-specific membrane antigen (PSMA) targeted [18F]DCFPyL PET/CT scans of patients with biochemical recurrence of prostate cancer, training deep neural networks, U-Net, Attention U-Net and SegResNet with four loss functions: Dice Loss, Dice Cross Entropy, Dice Focal Loss, and our proposed L1 weighted Dice Focal Loss (L1DFL). Evaluations indicated that Attention U-Net paired with L1DFL achieved the strongest correlation with the ground truth (concordance correlation = 0.90-0.99 for SUVmax and TLA), whereas models employing the Dice Loss and the other two compound losses, particularly with SegResNet, underperformed. Equivalence testing (TOST, alpha = 0.05, Delta = 20%) confirmed high performance for SUV metrics, lesion count and TLA, with L1DFL yielding the best performance. By contrast, tumor volume and lesion spread exhibited greater variability. Bland-Altman, Coverage Probability, and Total Deviation Index analyses further highlighted that our proposed L1DFL minimizes variability in quantification of the ground truth clinical measures. The code is publicly available at: https://github.com/ObedDzik/pca\_segment.git.
* 12 pages, 8 figures
Via
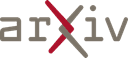
Apr 18, 2025
Abstract:Cancer detection and prognosis relies heavily on medical imaging, particularly CT and PET scans. Deep Neural Networks (DNNs) have shown promise in tumor segmentation by fusing information from these modalities. However, a critical bottleneck exists: the dependency on CT-PET data concurrently for training and inference, posing a challenge due to the limited availability of PET scans. Hence, there is a clear need for a flexible and efficient framework that can be trained with the widely available CT scans and can be still adapted for PET scans when they become available. In this work, we propose a parameter-efficient multi-modal adaptation (PEMMA) framework for lightweight upgrading of a transformer-based segmentation model trained only on CT scans such that it can be efficiently adapted for use with PET scans when they become available. This framework is further extended to perform prognosis task maintaining the same efficient cross-modal fine-tuning approach. The proposed approach is tested with two well-known segementation backbones, namely UNETR and Swin UNETR. Our approach offers two main advantages. Firstly, we leverage the inherent modularity of the transformer architecture and perform low-rank adaptation (LoRA) as well as decomposed low-rank adaptation (DoRA) of the attention weights to achieve parameter-efficient adaptation. Secondly, by minimizing cross-modal entanglement, PEMMA allows updates using only one modality without causing catastrophic forgetting in the other. Our method achieves comparable performance to early fusion, but with only 8% of the trainable parameters, and demonstrates a significant +28% Dice score improvement on PET scans when trained with a single modality. Furthermore, in prognosis, our method improves the concordance index by +10% when adapting a CT-pretrained model to include PET scans, and by +23% when adapting for both PET and EHR data.
Via
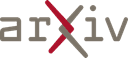
Apr 16, 2025
Abstract:Reliable tumor segmentation in thoracic computed tomography (CT) remains challenging due to boundary ambiguity, class imbalance, and anatomical variability. We propose an uncertainty-guided, coarse-to-fine segmentation framework that combines full-volume tumor localization with refined region-of-interest (ROI) segmentation, enhanced by anatomically aware post-processing. The first-stage model generates a coarse prediction, followed by anatomically informed filtering based on lung overlap, proximity to lung surfaces, and component size. The resulting ROIs are segmented by a second-stage model trained with uncertainty-aware loss functions to improve accuracy and boundary calibration in ambiguous regions. Experiments on private and public datasets demonstrate improvements in Dice and Hausdorff scores, with fewer false positives and enhanced spatial interpretability. These results highlight the value of combining uncertainty modeling and anatomical priors in cascaded segmentation pipelines for robust and clinically meaningful tumor delineation. On the Orlando dataset, our framework improved Swin UNETR Dice from 0.4690 to 0.6447. Reduction in spurious components was strongly correlated with segmentation gains, underscoring the value of anatomically informed post-processing.
* 6 pages, 2 figures, to appear in IEEE ADSCA 2025
Via
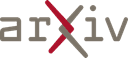
Apr 16, 2025
Abstract:Despite continuous advancements in cancer treatment, brain metastatic disease remains a significant complication of primary cancer and is associated with an unfavorable prognosis. One approach for improving diagnosis, management, and outcomes is to implement algorithms based on artificial intelligence for the automated segmentation of both pre- and post-treatment MRI brain images. Such algorithms rely on volumetric criteria for lesion identification and treatment response assessment, which are still not available in clinical practice. Therefore, it is critical to establish tools for rapid volumetric segmentations methods that can be translated to clinical practice and that are trained on high quality annotated data. The BraTS-METS 2025 Lighthouse Challenge aims to address this critical need by establishing inter-rater and intra-rater variability in dataset annotation by generating high quality annotated datasets from four individual instances of segmentation by neuroradiologists while being recorded on video (two instances doing "from scratch" and two instances after AI pre-segmentation). This high-quality annotated dataset will be used for testing phase in 2025 Lighthouse challenge and will be publicly released at the completion of the challenge. The 2025 Lighthouse challenge will also release the 2023 and 2024 segmented datasets that were annotated using an established pipeline of pre-segmentation, student annotation, two neuroradiologists checking, and one neuroradiologist finalizing the process. It builds upon its previous edition by including post-treatment cases in the dataset. Using these high-quality annotated datasets, the 2025 Lighthouse challenge plans to test benchmark algorithms for automated segmentation of pre-and post-treatment brain metastases (BM), trained on diverse and multi-institutional datasets of MRI images obtained from patients with brain metastases.
* 28 pages, 4 figures, 2 tables
Via
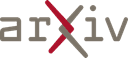
Apr 14, 2025
Abstract:Cancer remains one of the leading causes of mortality worldwide, and among its many forms, brain tumors are particularly notorious due to their aggressive nature and the critical challenges involved in early diagnosis. Recent advances in artificial intelligence have shown great promise in assisting medical professionals with precise tumor segmentation, a key step in timely diagnosis and treatment planning. However, many state-of-the-art segmentation methods require extensive computational resources and prolonged training times, limiting their practical application in resource-constrained settings. In this work, we present a novel dual-decoder U-Net architecture enhanced with attention-gated skip connections, designed specifically for brain tumor segmentation from MRI scans. Our approach balances efficiency and accuracy by achieving competitive segmentation performance while significantly reducing training demands. Evaluated on the BraTS 2020 dataset, the proposed model achieved Dice scores of 85.06% for Whole Tumor (WT), 80.61% for Tumor Core (TC), and 71.26% for Enhancing Tumor (ET) in only 50 epochs, surpassing several commonly used U-Net variants. Our model demonstrates that high-quality brain tumor segmentation is attainable even under limited computational resources, thereby offering a viable solution for researchers and clinicians operating with modest hardware. This resource-efficient model has the potential to improve early detection and diagnosis of brain tumors, ultimately contributing to better patient outcomes
Via
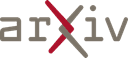
Apr 16, 2025
Abstract:Diffusion Probabilistic Models (DPMs) have demonstrated significant potential in 3D medical image segmentation tasks. However, their high computational cost and inability to fully capture global 3D contextual information limit their practical applications. To address these challenges, we propose a novel text-guided diffusion model framework, TextDiffSeg. This method leverages a conditional diffusion framework that integrates 3D volumetric data with natural language descriptions, enabling cross-modal embedding and establishing a shared semantic space between visual and textual modalities. By enhancing the model's ability to recognize complex anatomical structures, TextDiffSeg incorporates innovative label embedding techniques and cross-modal attention mechanisms, effectively reducing computational complexity while preserving global 3D contextual integrity. Experimental results demonstrate that TextDiffSeg consistently outperforms existing methods in segmentation tasks involving kidney and pancreas tumors, as well as multi-organ segmentation scenarios. Ablation studies further validate the effectiveness of key components, highlighting the synergistic interaction between text fusion, image feature extractor, and label encoder. TextDiffSeg provides an efficient and accurate solution for 3D medical image segmentation, showcasing its broad applicability in clinical diagnosis and treatment planning.
Via
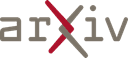
Apr 12, 2025
Abstract:Due to the success of CNN-based and Transformer-based models in various computer vision tasks, recent works study the applicability of CNN-Transformer hybrid architecture models in 3D multi-modality medical segmentation tasks. Introducing Transformer brings long-range dependent information modeling ability in 3D medical images to hybrid models via the self-attention mechanism. However, these models usually employ fixed receptive fields of 3D volumetric features within each self-attention layer, ignoring the multi-scale volumetric lesion features. To address this issue, we propose a CNN-Transformer hybrid 3D medical image segmentation model, named TMA-TransBTS, based on an encoder-decoder structure. TMA-TransBTS realizes simultaneous extraction of multi-scale 3D features and modeling of long-distance dependencies by multi-scale division and aggregation of 3D tokens in a self-attention layer. Furthermore, TMA-TransBTS proposes a 3D multi-scale cross-attention module to establish a link between the encoder and the decoder for extracting rich volume representations by exploiting the mutual attention mechanism of cross-attention and multi-scale aggregation of 3D tokens. Extensive experimental results on three public 3D medical segmentation datasets show that TMA-TransBTS achieves higher averaged segmentation results than previous state-of-the-art CNN-based 3D methods and CNN-Transform hybrid 3D methods for the segmentation of 3D multi-modality brain tumors.
Via
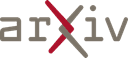
Apr 13, 2025
Abstract:Accurate segmentation of longitudinal CT scans is important for monitoring tumor progression and evaluating treatment responses. However, existing 3D segmentation models solely focus on spatial information. To address this gap, we propose OmniMamba4D, a novel segmentation model designed for 4D medical images (3D images over time). OmniMamba4D utilizes a spatio-temporal tetra-orientated Mamba block to effectively capture both spatial and temporal features. Unlike traditional 3D models, which analyze single-time points, OmniMamba4D processes 4D CT data, providing comprehensive spatio-temporal information on lesion progression. Evaluated on an internal dataset comprising of 3,252 CT scans, OmniMamba4D achieves a competitive Dice score of 0.682, comparable to state-of-the-arts (SOTA) models, while maintaining computational efficiency and better detecting disappeared lesions. This work demonstrates a new framework to leverage spatio-temporal information for longitudinal CT lesion segmentation.
* Accepted at IEEE International Symposium on Biomedical Imaging (ISBI)
2025
Via
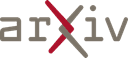
Apr 10, 2025
Abstract:Magnetic resonance imaging (MRI) raw data, or k-Space data, is complex-valued, containing both magnitude and phase information. However, clinical and existing Artificial Intelligence (AI)-based methods focus only on magnitude images, discarding the phase data despite its potential for downstream tasks, such as tumor segmentation and classification. In this work, we introduce $\textit{PhaseGen}$, a novel complex-valued diffusion model for generating synthetic MRI raw data conditioned on magnitude images, commonly used in clinical practice. This enables the creation of artificial complex-valued raw data, allowing pretraining for models that require k-Space information. We evaluate PhaseGen on two tasks: skull-stripping directly in k-Space and MRI reconstruction using the publicly available FastMRI dataset. Our results show that training with synthetic phase data significantly improves generalization for skull-stripping on real-world data, with an increased segmentation accuracy from $41.1\%$ to $80.1\%$, and enhances MRI reconstruction when combined with limited real-world data. This work presents a step forward in utilizing generative AI to bridge the gap between magnitude-based datasets and the complex-valued nature of MRI raw data. This approach allows researchers to leverage the vast amount of avaliable image domain data in combination with the information-rich k-Space data for more accurate and efficient diagnostic tasks. We make our code publicly $\href{https://github.com/TIO-IKIM/PhaseGen}{\text{available here}}$.
Via
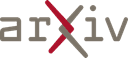