Topic:Traffic Sign Recognition
What is Traffic Sign Recognition? Traffic sign recognition is the process of identifying and categorizing different types of traffic signs in images or videos.
Papers and Code
Apr 13, 2025
Abstract:This study compares the performance of various preprocessing techniques for Traffic Sign Recognition (TSR) using Histogram of Oriented Gradients (HOG) and Support Vector Machine (SVM) on the German Traffic Sign Recognition Benchmark (GTSRB) dataset. Techniques such as CLAHE, HUE, and YUV were evaluated for their impact on classification accuracy. Results indicate that YUV in particular significantly enhance the performance of the HOG-SVM classifier (improving accuracy from 89.65% to 91.25%), providing insights into improvements for preprocessing pipeline of TSR applications.
* working paper (preprint)
Via
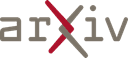
Mar 24, 2025
Abstract:The robustness of image recognition algorithms remains a critical challenge, as current models often depend on large quantities of labeled data. In this paper, we propose a hybrid approach that combines the adaptability of neural networks with the interpretability, transparency, and robustness of domain-specific quasi-invariant operators. Our method decomposes the recognition into multiple task-specific operators that focus on different characteristics, supported by a novel confidence measurement tailored to these operators. This measurement enables the network to prioritize reliable features and accounts for noise. We argue that our design enhances transparency and robustness, leading to improved performance, particularly in low-data regimes. Experimental results in traffic sign detection highlight the effectiveness of the proposed method, especially in semi-supervised and unsupervised scenarios, underscoring its potential for data-constrained applications.
Via
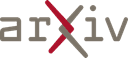
Mar 08, 2025
Abstract:Autonomous vehicles (AVs) require reliable traffic sign recognition and robust lane detection capabilities to ensure safe navigation in complex and dynamic environments. This paper introduces an integrated approach combining advanced deep learning techniques and Multimodal Large Language Models (MLLMs) for comprehensive road perception. For traffic sign recognition, we systematically evaluate ResNet-50, YOLOv8, and RT-DETR, achieving state-of-the-art performance of 99.8% with ResNet-50, 98.0% accuracy with YOLOv8, and achieved 96.6% accuracy in RT-DETR despite its higher computational complexity. For lane detection, we propose a CNN-based segmentation method enhanced by polynomial curve fitting, which delivers high accuracy under favorable conditions. Furthermore, we introduce a lightweight, Multimodal, LLM-based framework that directly undergoes instruction tuning using small yet diverse datasets, eliminating the need for initial pretraining. This framework effectively handles various lane types, complex intersections, and merging zones, significantly enhancing lane detection reliability by reasoning under adverse conditions. Despite constraints in available training resources, our multimodal approach demonstrates advanced reasoning capabilities, achieving a Frame Overall Accuracy (FRM) of 53.87%, a Question Overall Accuracy (QNS) of 82.83%, lane detection accuracies of 99.6% in clear conditions and 93.0% at night, and robust performance in reasoning about lane invisibility due to rain (88.4%) or road degradation (95.6%). The proposed comprehensive framework markedly enhances AV perception reliability, thus contributing significantly to safer autonomous driving across diverse and challenging road scenarios.
* 11 pages, 9 figures
Via
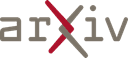
Feb 27, 2025
Abstract:Adversarial attacks on machine learning models often rely on small, imperceptible perturbations to mislead classifiers. Such strategy focuses on minimizing the visual perturbation for humans so they are not confused, and also maximizing the misclassification for machine learning algorithms. An orthogonal strategy for adversarial attacks is to create perturbations that are clearly visible but do not confuse humans, yet still maximize misclassification for machine learning algorithms. This work follows the later strategy, and demonstrates instance of it through the Snowball Adversarial Attack in the context of traffic sign recognition. The attack leverages the human brain's superior ability to recognize objects despite various occlusions, while machine learning algorithms are easily confused. The evaluation shows that the Snowball Adversarial Attack is robust across various images and is able to confuse state-of-the-art traffic sign recognition algorithm. The findings reveal that Snowball Adversarial Attack can significantly degrade model performance with minimal effort, raising important concerns about the vulnerabilities of deep neural networks and highlighting the necessity for improved defenses for image recognition machine learning models.
Via
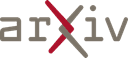
Feb 21, 2025
Abstract:Traffic signs recognition (TSR) plays an essential role in assistant driving and intelligent transportation system. However, the noise of complex environment may lead to motion-blur or occlusion problems, which raise the tough challenge to real-time recognition with high accuracy and robust. In this article, we propose IECES-network which with improved encoders and Siamese net. The three-stage approach of our method includes Efficient-CNN based encoders, Siamese backbone and the fully-connected layers. We firstly use convolutional encoders to extract and encode the traffic sign features of augmented training samples and standard images. Then, we design the Siamese neural network with Efficient-CNN based encoder and contrastive loss function, which can be trained to improve the robustness of TSR problem when facing the samples of motion-blur and occlusion by computing the distance between inputs and templates. Additionally, the template branch of the proposed network can be stopped when executing the recognition tasks after training to raise the process speed of our real-time model, and alleviate the computational resource and parameter scale. Finally, we recombined the feature code and a fully-connected layer with SoftMax function to classify the codes of samples and recognize the category of traffic signs. The results of experiments on the Tsinghua-Tencent 100K dataset and the German Traffic Sign Recognition Benchmark dataset demonstrate the performance of the proposed IECESnetwork. Compared with other state-of-the-art methods, in the case of motion-blur and occluded environment, the proposed method achieves competitive performance precision-recall and accuracy metric average is 88.1%, 86.43% and 86.1% with a 2.9M lightweight scale, respectively. Moreover, processing time of our model is 0.1s per frame, of which the speed is increased by 1.5 times compared with existing methods.
Via
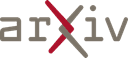
Feb 26, 2025
Abstract:Adversarial attacks on deep learning models have proliferated in recent years. In many cases, a different adversarial perturbation is required to be added to each image to cause the deep learning model to misclassify it. This is ineffective as each image has to be modified in a different way. Meanwhile, research on universal perturbations focuses on designing a single perturbation that can be applied to all images in a data set, and cause a deep learning model to misclassify the images. This work advances the field of universal perturbations by exploring universal perturbations in the context of traffic signs and autonomous vehicle systems. This work introduces a novel method for generating universal perturbations that visually look like simple black and white stickers, and using them to cause incorrect street sign predictions. Unlike traditional adversarial perturbations, the adversarial universal stickers are designed to be applicable to any street sign: same sticker, or stickers, can be applied in same location to any street sign and cause it to be misclassified. Further, to enable safe experimentation with adversarial images and street signs, this work presents a virtual setting that leverages Street View images of street signs, rather than the need to physically modify street signs, to test the attacks. The experiments in the virtual setting demonstrate that these stickers can consistently mislead deep learning models used commonly in street sign recognition, and achieve high attack success rates on dataset of US traffic signs. The findings highlight the practical security risks posed by simple stickers applied to traffic signs, and the ease with which adversaries can generate adversarial universal stickers that can be applied to many street signs.
Via
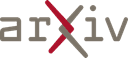
Jan 16, 2025
Abstract:We explore the impact of aperture size and shape on automotive camera systems for deep-learning-based tasks like traffic sign recognition and light state detection. A method is proposed to simulate optical effects using the point spread function (PSF), enhancing realism and reducing the domain gap between synthetic and real-world images. Computer-generated scenes are refined with this technique to model optical distortions and improve simulation accuracy.
Via
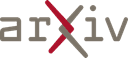
Jan 14, 2025
Abstract:The deployment of neural networks in vehicle platforms and wearable Artificial Intelligence-of-Things (AIOT) scenarios has become a research area that has attracted much attention. With the continuous evolution of deep learning technology, many image classification models are committed to improving recognition accuracy, but this is often accompanied by problems such as large model resource usage, complex structure, and high power consumption, which makes it challenging to deploy on resource-constrained platforms. Herein, we propose an ultra-lightweight binary neural network (BNN) model designed for hardware deployment, and conduct image classification research based on the German Traffic Sign Recognition Benchmark (GTSRB) dataset. In addition, we also verify it on the Chinese Traffic Sign (CTS) and Belgian Traffic Sign (BTS) datasets. The proposed model shows excellent recognition performance with an accuracy of up to 97.64%, making it one of the best performing BNN models in the GTSRB dataset. Compared with the full-precision model, the accuracy loss is controlled within 1%, and the parameter storage overhead of the model is only 10% of that of the full-precision model. More importantly, our network model only relies on logical operations and low-bit width fixed-point addition and subtraction operations during the inference phase, which greatly simplifies the design complexity of the processing element (PE). Our research shows the great potential of BNN in the hardware deployment of computer vision models, especially in the field of computer vision tasks related to autonomous driving.
Via
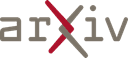
Jan 08, 2025
Abstract:Intelligent Transportation Systems (ITS) are crucial for the development and operation of smart cities, addressing key challenges in efficiency, productivity, and environmental sustainability. This paper comprehensively reviews the transformative potential of Large Language Models (LLMs) in optimizing ITS. Initially, we provide an extensive overview of ITS, highlighting its components, operational principles, and overall effectiveness. We then delve into the theoretical background of various LLM techniques, such as GPT, T5, CTRL, and BERT, elucidating their relevance to ITS applications. Following this, we examine the wide-ranging applications of LLMs within ITS, including traffic flow prediction, vehicle detection and classification, autonomous driving, traffic sign recognition, and pedestrian detection. Our analysis reveals how these advanced models can significantly enhance traffic management and safety. Finally, we explore the challenges and limitations LLMs face in ITS, such as data availability, computational constraints, and ethical considerations. We also present several future research directions and potential innovations to address these challenges. This paper aims to guide researchers and practitioners through the complexities and opportunities of integrating LLMs in ITS, offering a roadmap to create more efficient, sustainable, and responsive next-generation transportation systems.
* Accepted for publication in IEEE Transactions on Intelligent
Transportation Systems
Via
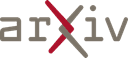
Dec 29, 2024
Abstract:Operational machine-learning based assistant systems must be robust in a wide range of scenarios. This hold especially true for the air-traffic control (ATC) domain. The robustness of an architecture is particularly evident in edge cases, such as high word error rate (WER) transcripts resulting from noisy ATC recordings or partial transcripts due to clipped recordings. To increase the edge-case robustness of call-sign recognition and understanding (CRU), a core tasks in ATC speech processing, we propose the multimodal call-sign-command recovery model (CCR). The CCR architecture leads to an increase in the edge case performance of up to 15%. We demonstrate this on our second proposed architecture, CallSBERT. A CRU model that has less parameters, can be fine-tuned noticeably faster and is more robust during fine-tuning than the state of the art for CRU. Furthermore, we demonstrate that optimizing for edge cases leads to a significantly higher accuracy across a wide operational range.
Via
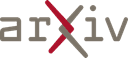