What is Cantilever Beam? A cantilever beam is a beam that is supported at one end and free at the other end, allowing it to carry loads and resist bending.
Papers and Code
Mar 25, 2025
Abstract:Surrogate models are of high interest for many engineering applications, serving as cheap-to-evaluate time-efficient approximations of black-box functions to help engineers and practitioners make decisions and understand complex systems. As such, the need for explainability methods is rising and many studies have been performed to facilitate knowledge discovery from surrogate models. To respond to these enquiries, this paper introduces SMT-EX, an enhancement of the open-source Python Surrogate Modeling Toolbox (SMT) that integrates explainability techniques into a state-of-the-art surrogate modelling framework. More precisely, SMT-EX includes three key explainability methods: Shapley Additive Explanations, Partial Dependence Plot, and Individual Conditional Expectations. A peculiar explainability dependency of SMT has been developed for such purpose that can be easily activated once the surrogate model is built, offering a user-friendly and efficient tool for swift insight extraction. The effectiveness of SMT-EX is showcased through two test cases. The first case is a 10-variable wing weight problem with purely continuous variables and the second one is a 3-variable mixed-categorical cantilever beam bending problem. Relying on SMT-EX analyses for these problems, we demonstrate its versatility in addressing a diverse range of problem characteristics. SMT-Explainability is freely available on Github: https://github.com/SMTorg/smt-explainability .
* Robani, M. D., Saves, P., Palar, P. S., Zuhal, L. R., & Morlier,
J. (2025). SMT-EX: An Explainable Surrogate Modeling Toolbox for
Mixed-Variables Design Exploration. In AIAA SCITECH 2025 Forum (p. 0777)
Via
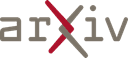
Jul 15, 2024
Abstract:Artificial intelligence and machine learning frameworks have served as computationally efficient mapping between inputs and outputs for engineering problems. These mappings have enabled optimization and analysis routines that have warranted superior designs, ingenious material systems and optimized manufacturing processes. A common occurrence in such modeling endeavors is the existence of multiple source of data, each differentiated by fidelity, operating conditions, experimental conditions, and more. Data fusion frameworks have opened the possibility of combining such differentiated sources into single unified models, enabling improved accuracy and knowledge transfer. However, these frameworks encounter limitations when the different sources are heterogeneous in nature, i.e., not sharing the same input parameter space. These heterogeneous input scenarios can occur when the domains differentiated by complexity, scale, and fidelity require different parametrizations. Towards addressing this void, a heterogeneous multi-source data fusion framework is proposed based on input mapping calibration (IMC) and latent variable Gaussian process (LVGP). In the first stage, the IMC algorithm is utilized to transform the heterogeneous input parameter spaces into a unified reference parameter space. In the second stage, a multi-source data fusion model enabled by LVGP is leveraged to build a single source-aware surrogate model on the transformed reference space. The proposed framework is demonstrated and analyzed on three engineering case studies (design of cantilever beam, design of ellipsoidal void and modeling properties of Ti6Al4V alloy). The results indicate that the proposed framework provides improved predictive accuracy over a single source model and transformed but source unaware model.
* 20 Pages,9 Figures, Data is available per request
Via
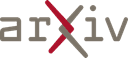
Jun 23, 2024
Abstract:This paper investigates the optimization of 2D and 3D composite structures using machine learning (ML) techniques, focusing on fracture toughness and crack propagation in the Double Cantilever Beam (DCB) test. By exploring the intricate relationship between microstructural arrangements and macroscopic properties of composites, the study demonstrates the potential of ML as a powerful tool to expedite the design optimization process, offering notable advantages over traditional finite element analysis. The research encompasses four distinct cases, examining crack propagation and fracture toughness in both 2D and 3D composite models. Through the application of ML algorithms, the study showcases the capability for rapid and accurate exploration of vast design spaces in composite materials. The findings highlight the efficiency of ML in predicting mechanical behaviors with limited training data, paving the way for broader applications in composite design and optimization. This work contributes to advancing the understanding of ML's role in enhancing the efficiency of composite material design processes.
Via
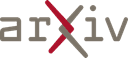
Feb 21, 2024
Abstract:Sea Horse Optimizer (SHO) is a noteworthy metaheuristic algorithm that emulates various intelligent behaviors exhibited by sea horses, encompassing feeding patterns, male reproductive strategies, and intricate movement patterns. To mimic the nuanced locomotion of sea horses, SHO integrates the logarithmic helical equation and Levy flight, effectively incorporating both random movements with substantial step sizes and refined local exploitation. Additionally, the utilization of Brownian motion facilitates a more comprehensive exploration of the search space. This study introduces a robust and high-performance variant of the SHO algorithm named mSHO. The enhancement primarily focuses on bolstering SHO's exploitation capabilities by replacing its original method with an innovative local search strategy encompassing three distinct steps: a neighborhood-based local search, a global non-neighbor-based search, and a method involving circumnavigation of the existing search region. These techniques improve mSHO algorithm's search capabilities, allowing it to navigate the search space and converge toward optimal solutions efficiently. The comprehensive results distinctly establish the supremacy and efficiency of the mSHO method as an exemplary tool for tackling an array of optimization quandaries. The results show that the proposed mSHO algorithm has a total rank of 1 for CEC'2020 test functions. In contrast, the mSHO achieved the best value for the engineering problems, recording a value of 0.012665, 2993.634, 0.01266, 1.724967, 263.8915, 0.032255, 58507.14, 1.339956, and 0.23524 for the pressure vessel design, speed reducer design, tension/compression spring, welded beam design, three-bar truss engineering design, industrial refrigeration system, multi-Product batch plant, cantilever beam problem, multiple disc clutch brake problems, respectively.
Via
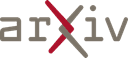
Nov 10, 2023
Abstract:Multidisciplinary design optimization (MDO) methods aim at adapting numerical optimization techniques to the design of engineering systems involving multiple disciplines. In this context, a large number of mixed continuous, integer, and categorical variables might arise during the optimization process, and practical applications involve a significant number of design variables. Recently, there has been a growing interest in mixed-categorical metamodels based on Gaussian Process (GP) for Bayesian optimization. In particular, to handle mixed-categorical variables, several existing approaches employ different strategies to build the GP. These strategies either use continuous kernels, such as the continuous relaxation or the Gower distance-based kernels, or direct estimation of the correlation matrix, such as the exponential homoscedastic hypersphere (EHH) or the Homoscedastic Hypersphere (HH) kernel. Although the EHH and HH kernels are shown to be very efficient and lead to accurate GPs, they are based on a large number of hyperparameters. In this paper, we address this issue by constructing mixed-categorical GPs with fewer hyperparameters using Partial Least Squares (PLS) regression. Our goal is to generalize Kriging with PLS, commonly used for continuous inputs, to handle mixed-categorical inputs. The proposed method is implemented in the open-source software SMT and has been efficiently applied to structural and multidisciplinary applications. Our method is used to effectively demonstrate the structural behavior of a cantilever beam and facilitates MDO of a green aircraft, resulting in a 439-kilogram reduction in the amount of fuel consumed during a single aircraft mission.
Via
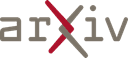
Oct 19, 2023
Abstract:Soft pneumatic actuators (SPAs) are widely employed to drive soft robots. However, their inherent flexibility offers both benefits and challenges. This property reduces their output force/torque and makes them hard to control. This paper introduces a new design method that enhances the actuator's performance and controllability. The complex structure of the soft actuator is simplified by approximating it as a cantilever beam. This allows us to derive a mechanical equation between input pressure to output torque. Additionally, a dynamical model is explored to understand the correlation between the natural frequency and dimensional parameters of the SPA. The design problem is then transformed into an optimization problem, using the mechanical equation as the objective function and the dynamical equation as a constraint. By solving this optimization problem, the optimal dimensional parameters are determined. Prior to fabrication, preliminary tests are conducted using the finite element method. Six prototypes are manufactured to validate the proposed approach. The optimal actuator successfully generates the desired force/torque, while its natural frequency remains within the constrained range. This work highlights the potential of using approximated models and optimization formulation to boost the efficiency and dynamic performance of soft pneumatic actuators.
* 11 pages, 11 figures
Via
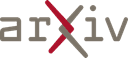
Sep 20, 2023
Abstract:In this article, we propose a new metaheuristic inspired by the morphogenetic cellular movements of endothelial cells (ECs) that occur during the tumor angiogenesis process. This algorithm starts with a random initial population. In each iteration, the best candidate selected as the tumor, while the other individuals in the population are treated as ECs migrating toward the tumor's direction following a coordinated dynamics through a spatial relationship between tip and follower ECs. This algorithm has an advantage compared to other similar optimization metaheuristics: the model parameters are already configured according to the tumor angiogenesis phenomenon modeling, preventing researchers from initializing them with arbitrary values. Subsequently, the algorithm is compared against well-known benchmark functions, and the results are validated through a comparative study with Particle Swarm Optimization (PSO). The results demonstrate that the algorithm is capable of providing highly competitive outcomes. Furthermore, the proposed algorithm is applied to real-world problems (cantilever beam design, pressure vessel design, tension/compression spring and sustainable explotation renewable resource). The results showed that the proposed algorithm worked effectively in solving constrained optimization problems. The results obtained were compared with several known algorithms.
* 16 pages; 7 figures; added real-world problems in section 4 (pressure
vessel design, tension/compression spring and sustainable explotation
renewable resource); added references for section 4; typos corrected
Via
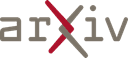
Aug 05, 2023
Abstract:A physics-informed machine learning model, in the form of a multi-output Gaussian process, is formulated using the Euler-Bernoulli beam equation. Given appropriate datasets, the model can be used to regress the analytical value of the structure's bending stiffness, interpolate responses, and make probabilistic inferences on latent physical quantities. The developed model is applied on a numerically simulated cantilever beam, where the regressed bending stiffness is evaluated and the influence measurement noise on the prediction quality is investigated. Further, the regressed probabilistic stiffness distribution is used in a structural health monitoring context, where the Mahalanobis distance is employed to reason about the possible location and extent of damage in the structural system. To validate the developed framework, an experiment is conducted and measured heterogeneous datasets are used to update the assumed analytical structural model.
* IABSE Symposium: Challenges for Existing and Oncoming Structures,
Prague, Czech Republic, 25-27 May 2022
* 10 pages, 10 figures, 11 references
Via
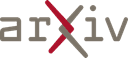
Aug 02, 2023
Abstract:The digital twin concept represents an appealing opportunity to advance condition-based and predictive maintenance paradigms for civil engineering systems, thus allowing reduced lifecycle costs, increased system safety, and increased system availability. This work proposes a predictive digital twin approach to the health monitoring, maintenance, and management planning of civil engineering structures. The asset-twin coupled dynamical system is encoded employing a probabilistic graphical model, which allows all relevant sources of uncertainty to be taken into account. In particular, the time-repeating observations-to-decisions flow is modeled using a dynamic Bayesian network. Real-time structural health diagnostics are provided by assimilating sensed data with deep learning models. The digital twin state is continually updated in a sequential Bayesian inference fashion. This is then exploited to inform the optimal planning of maintenance and management actions within a dynamic decision-making framework. A preliminary offline phase involves the population of training datasets through a reduced-order numerical model and the computation of a health-dependent control policy. The strategy is assessed on two synthetic case studies, involving a cantilever beam and a railway bridge, demonstrating the dynamic decision-making capabilities of health-aware digital twins.
Via
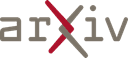
May 04, 2023
Abstract:In the last decade, damage detection approaches swiftly changed from advanced signal processing methods to machine learning and especially deep learning models, to accurately and non-intrusively estimate the state of the beam structures. But as the deep learning models reached their peak performances, also their limitations in applicability and vulnerabilities were observed. One of the most important reason for the lack of trustworthiness in operational conditions is the absence of intrinsic explainability of the deep learning system, due to the encoding of the knowledge in tensor values and without the inclusion of logical constraints. In this paper, we propose a neuro-symbolic model for the detection of damages in cantilever beams based on a novel cognitive architecture in which we join the processing power of convolutional networks with the interactive control offered by queries realized through the inclusion of real logic directly into the model. The hybrid discriminative model is introduced under the name Logic Convolutional Neural Regressor and it is tested on a dataset of values of the relative natural frequency shifts of cantilever beams derived from an original mathematical relation. While the obtained results preserve all the predictive capabilities of deep learning models, the usage of three distances as predicates for satisfiability, makes the system more trustworthy and scalable for practical applications. Extensive numerical and laboratory experiments were performed, and they all demonstrated the superiority of the hybrid approach, which can open a new path for solving the damage detection problem.
Via
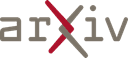