Mar 11, 2023
Abstract:A univariate time series with high variability can pose a challenge even to Deep Neural Network (DNN). To overcome this, a univariate time series is decomposed into simpler constituent series, whose sum equals the original series. As demonstrated in this article, the conventional one-time decomposition technique suffers from a leak of information from the future, referred to as a data leak. In this work, a novel Moving Front (MF) method is proposed to prevent data leakage, so that the decomposed series can be treated like other time series. Indian Summer Monsoon Rainfall (ISMR) is a very complex time series, which poses a challenge to DNN and is therefore selected as an example. From the many signal processing tools available, Empirical Wavelet Transform (EWT) was chosen for decomposing the ISMR into simpler constituent series, as it was found to be more effective than the other popular algorithm, Complete Ensemble Empirical Mode Decomposition with Adaptive Noise (CEEMDAN). The proposed MF method was used to generate the constituent leakage-free time series. Predictions and forecasts were made by state-of-the-art Long and Short-Term Memory (LSTM) network architecture, especially suitable for making predictions of sequential patterns. The constituent MF series has been divided into training, testing, and forecasting. It has been found that the model (EWT-MF-LSTM) developed here made exceptionally good train and test predictions, as well as Walk-Forward Validation (WFV), forecasts with Performance Parameter ($PP$) values of 0.99, 0.86, and 0.95, respectively, where $PP$ = 1.0 signifies perfect reproduction of the data.
Via
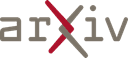